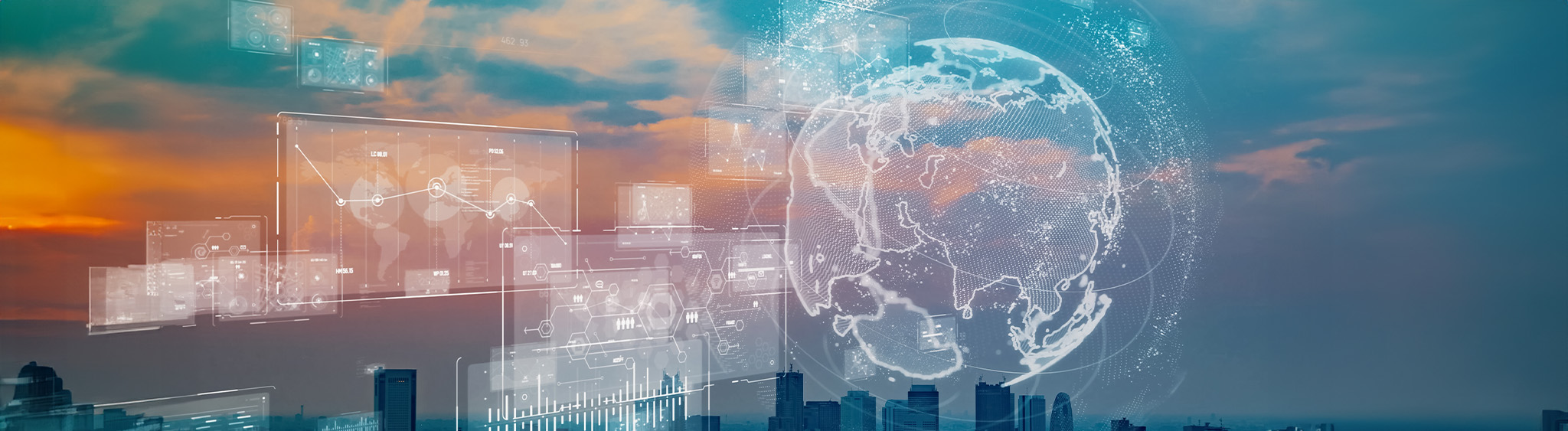
As Generative AI (gen AI) becomes a strategic priority, enterprises are racing to harness the power of Large Language Models (LLMs) for tasks like content creation, search, and automation. However, while LLMs are impressive, they’re not without limitations.
LLMs are also inherently stateless, which means that they don’t remember past queries and rely solely on their training data to generate responses. This often leads to generic or outdated answers, especially when users need timely, context-specific information.
This is where vector databases step in as a potential solution, as our analysts have highlighted below.
Reach out to discuss this topic in depth.
What is the role of vector databases in information retrieval?
Vector databases are purpose-built for contextual retrieval. Instead of storing data as text or rows and columns, they store vector embeddings, which are dense numerical representations that capture the meaning behind text, images, or audio.
Think of it like this: if you ask for movies like Inception, a traditional database may just look for the same title or keywords. A vector database, however, understands you’re looking for movies with similar themes: sci-fi thrillers, layered narratives, psychological twists, and delivers recommendations accordingly.
This ability to understand and retrieve information based on context is what makes vector databases impactful for artificial intelligence (AI)-driven applications.
What does vector information retrieval look like?
A typical vector information retrieval pipeline includes:
- Input query: User submits a question in natural language
- Vectorization: The query and data are transformed into numerical representations
- Indexing: Vectors are structured for efficient retrieval
- Query execution: A semantic search is performed to identify the most relevant matches
Exhibit 1: The main idea behind vector embeddings is to capture the semantic information of the data, such as its meaning, context, or similarity to other data points.
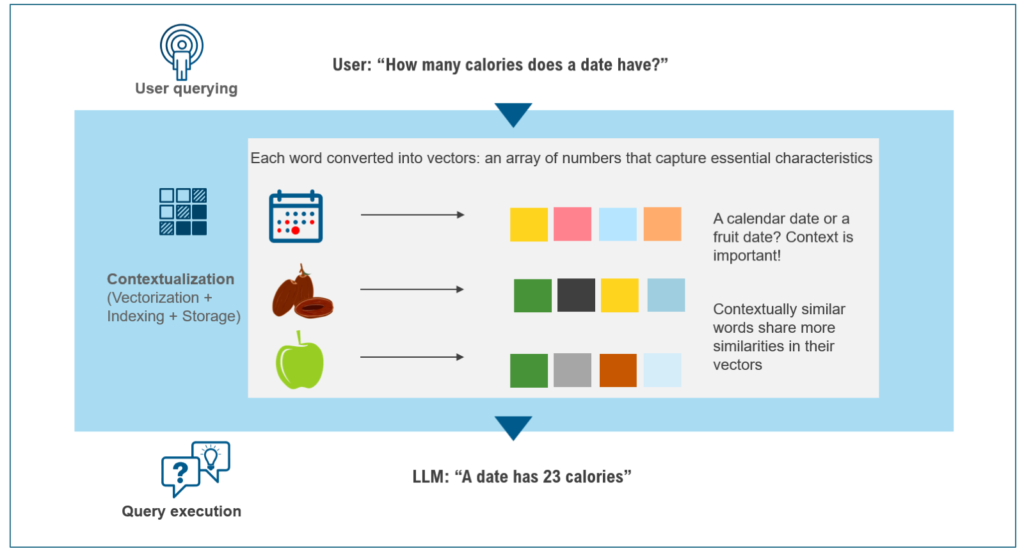
This vector-driven approach enables more flexible and intelligent retrieval, enabling use cases where traditional search fails, like personalized recommendations, conversational memory, or multi-modal search (text, image, audio).
In short, vector databases aren’t just a storage upgrade, they’re a fundamental enabler of next-gen information retrieval, enabling intelligent systems that can understand what you’re asking.
Powering the next generation of AI
Retrieval-Augmented Generation (RAG) is a powerful framework gaining traction across the AI community. It enhances LLMs by enabling them to access relevant external data in real time, resulting in responses that are more accurate, grounded, and up to date. This approach depends on having a strong retrieval mechanism in place, precisely the kind of capability vector-based systems are designed to deliver.
With RAG and vector databases working together, AI systems can deliver responses that are not just fluent, but also factual, personalized, and context aware. The synergy ensures that the most relevant pieces of information are identified and retrieved instantly, enriching LLM outputs with context. This combination is particularly powerful for dynamic, fast-changing domains where static knowledge simply isn’t enough.
We are already seeing some initial successes such as:
- Chatbots that provide relevant answers even in multi-turn conversations
- Search engines that understand intent and context
- Virtual assistants that adapt to individual users
- Fraud detection tools that surface subtle patterns in real-time
Despite its benefits, vector database adoption is still in its early stages. High implementation costs, limited awareness, and a lack of enterprise-ready tools have made enterprises hesitant in adopting vector databases.
Many enterprises are questioning if they really need yet another database tool in their already complicated stacks, when other databases can also provide vector search capabilities. But as more success stories emerge and tooling improves, vector databases are now likely to become the backbone of intelligent information retrieval in AI workflows.
Does your enterprise have vector search use cases?
Wondering if a vector database is the right fit for your AI initiatives?
Struggling to navigate a crowded vendor landscape?
Our upcoming thought leadership on vector databases will help you make the right decision.
If you found this blog interesting, check out our Navigating The Agentic AI Tech Landscape: Discovering The Ideal Strategic Partner | Blog – Everest Group, which delves deeper into another topic regarding AI.
Until then, let’s connect! Reach out to us with your questions and we’d be happy to chat: Abhigyan Malik ([email protected]), Mansi Gupta ([email protected]), Ishi Thakur ([email protected]).