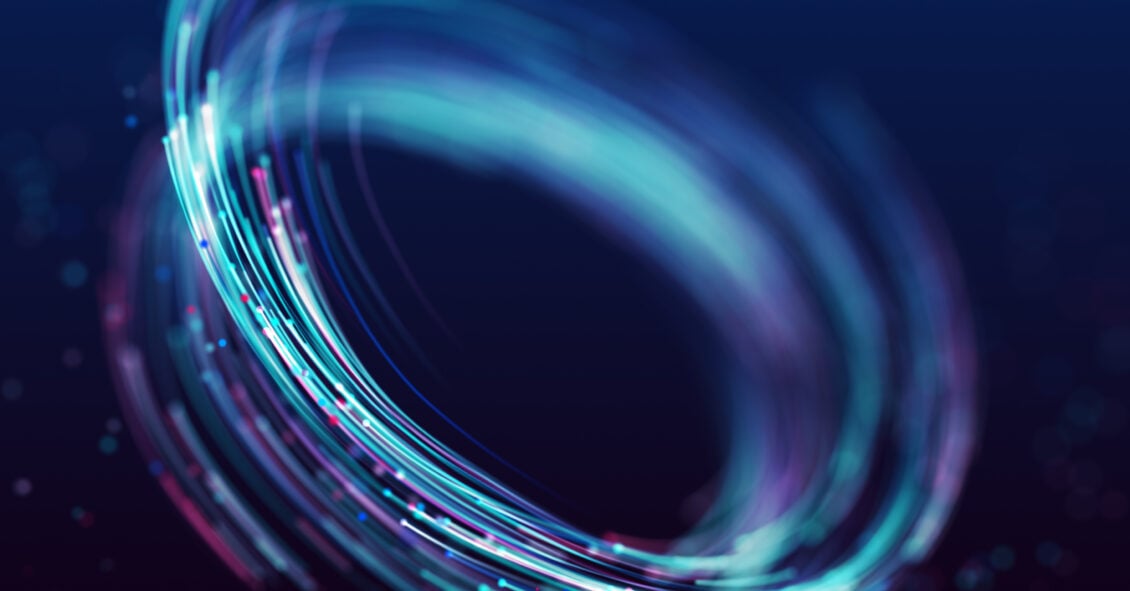
In the rapidly evolving world of automation, new concepts and buzzwords frequently spark excitement, anticipation, and sometimes confusion.
One such concept currently captivating the automation community is agentic AI. Many view agentic AI as the next transformative advancement beyond existing Intelligent Automation (IA) technologies. However, much of this excitement is clouded by misunderstandings about what agentic AI truly is and what it can achieve.
In this blog, we will clarify the concept of agentic AI, highlight how it differs from IA, and explain why it is such a significant leap forward for organizations poised to embrace the future of automation.
Reach out to discuss this topic in depth.
Understanding Agentic AI
First, let us define agentic AI. The term, agentic, refers to the idea of having the capacity for independent action and decision-making. Everest Group defines agentic AI as an evolved form of artificial intelligence (AI) that creates autonomous agents with high levels of autonomy, intelligence, decision-making, and adaptability. These agents can perform complex tasks based on simple and natural language inputs, including setting goals, planning, and taking actions in a dynamic environment with minimal human intervention.
Exhibit 1: Overview of agentic AI workflow
Source: Everest Group (2024)
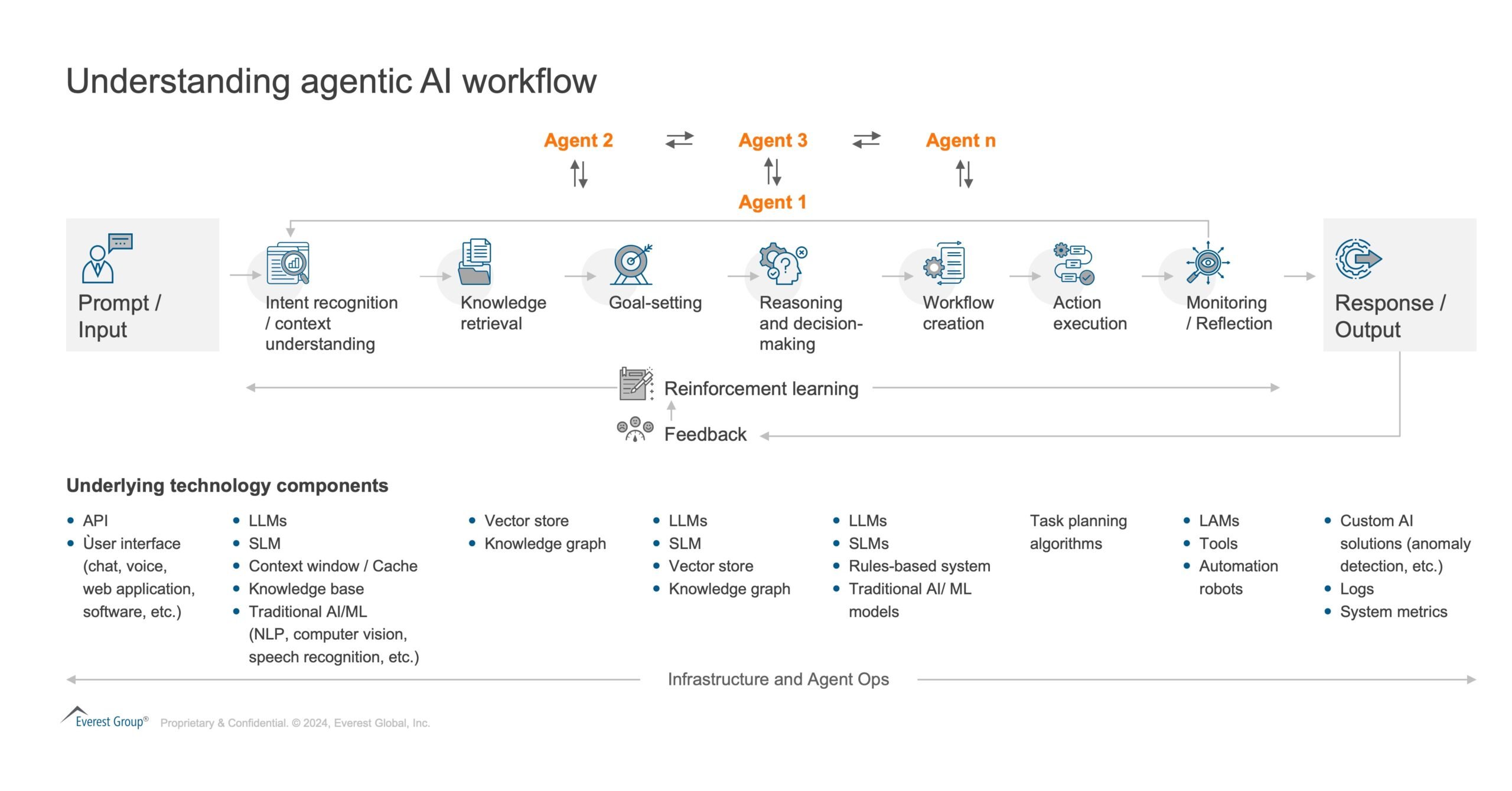
As illustrated in Exhibit 1, an agentic AI system goes through a series of steps to transform user inputs, into meaningful outputs, utilizing a range of technologies at each step.
- Upon receiving an input or trigger through sources such as voice, chat, web applications, or Application Programming Interfaces (APIs), the system employs both traditional AI techniques such as Natural Language Processing (NLP), as well as generative AI (gen AI) to accurately interpret user intent. Additionally, it retrieves essential supplementary information to enhance contextual understanding through short-and long-term memory such as context windows, vector stores, and knowledge graphs
- Based on user intent, the system breaks down task into goals, utilizing specialized models and knowledge management tools
- It then analyzes potential actions and outcomes by employing various rules-based and AI models. Once a decision path is established, the system creates workflow and executes action. Task planning algorithms and automation tools organize tasks into an actionable sequence, which is then executed by automation robots or other tools
- The system continuously monitors and reflects on its actions, incorporating reinforcement learning and feedback to improve accuracy and efficiency over time
Through this workflow, agents can operate autonomously while adapting to new inputs and challenges dynamically, making agentic AI a powerful technology for complex decision-making and automation. Agentic AI has the potential to significantly transform business operations, way beyond what traditional IA technologies can do. To better understand this advancement, let us first review the role of IA.
A quick recap of Intelligent Automation (IA): the overview, benefits, and limitations
IA automates business processes by using various technologies such as Robotic Process Automation (RPA), process orchestration, and cognitive/AI-based automation, including Conversational AI (CAI) and Intelligent Document Processing (IDP). These IA technologies offer scalable, efficient solutions for the automation of repetitive, high-volume tasks such as processing invoices, managing customer service inquiries, and handling payroll, hence freeing human workers to focus on strategic goals, and enabling organizations to scale cost-effectively. Additionally, IA enhances governance and compliance with automated tracking and reporting.
Through augmentation with deterministic AI in the last few years, IA has been able to incorporate a decent level of self-learning, intelligence, and decision making. However, IA still has its limitations. It works well for structured, repetitive, low to medium complexity tasks, but needs human intervention for more complex scenarios requiring flexibility, judgment, or innovation, because of which it often does not achieve end-to-end process automation. This is where agentic AI steps in.
The leap: Why agentic AI is a game-changer?
The leap from IA to agentic AI can be synonymous with moving from a basic autopilot system on an airplane, to one capable of navigating unpredictable turbulence or emergency rerouting – all without needing human input at every step. Both existing IA technologies and agentic AI, reduces human intervention and boosts productivity, however, they differ significantly in terms of complexity, autonomy, adaptability, and decision-making.
Let us examine the attributes that set agentic AI apart from existing IA technologies:
- Scope: Both traditional IA and agentic AI can be integrated into existing business processes, but IA primarily manages well-defined, repeatable tasks such as data extraction, customer interactions, and data analysis. Agentic AI, on the other hand, extends beyond simple tasks to strategic problem-solving in complex, uncertain environments. Its broader scope allows for dynamic adjustments, making it useful in use cases such as autonomous demand forecasting and adaptive supply chains
- Task vs. goal-orientation: IA technologies such as RPA, IDP, and CAI focus on predictable, task-specific outcomes, such as processing an invoice or generating a report. In contrast, agentic AI adopts a flexible, goal-oriented approach, pursuing broader objectives and adapting its strategies to achieve them. These goals, typically informed by user interactions and environmental conditions, guide the agent’s actions, allowing it to prioritize tasks dynamically to meet high-level objectives
- Autonomy in decision-making and human oversight: Traditional IA relies on rules-based decisioning and predefined workflows. In scenarios falling beyond its defined parameters, either it stops or escalates for human intervention. In contrast, agentic AI operates with a high degree of autonomy, making decisions and strategic actions based on real-time data, without human intervention. While some oversight remains necessary for high-stakes or ethical decisions, agentic AI minimizes the need for constant monitoring
- Multi-agent collaboration: In traditional IA constructs, robots or digital workers based on technologies such as RPA, IDP, and CAI collaborate through a process orchestration layer / solution based on predefined rules, often needing human intervention for validations and adjustments. Agentic AI, however, can coordinate multiple agents, often using traditional IA technologies as tools, to manage subtasks either in parallel or sequentially. These agents collaborate, negotiate, and delegate autonomously, enhancing dynamic and goal-oriented collaboration. For instance, during a sudden surge in customer complaints, IA would process them as per its original workflow. In an agentic AI system, one agent addresses initial queries and identifies their nature, while another analyzes sentiment. If negative sentiment increases, cases are dynamically assigned to additional support agents specialized in similar issues, improving response times and customer satisfaction
- Workflow optimization: IA technologies such as process orchestration aim for process optimization by relying on structured workflows and rules, which require specific triggers and structured inputs. Agentic AI, on the other hand, continuously analyzes and adjusts workflows in real time to achieve set goals with minimal delay and optimal resource efficiency
- Self-learning: IA technologies such as RPA lacks the capability for self-learning, while AI-based tools such as IDP and CAI require retraining by humans and cannot autonomously adjust based on evolving goals. In contrast, agentic AI evaluates its actions’ effectiveness, creates an iterative self-improvement cycle, and adjusts future behavior based on successes or failures. For example, it refines its collaboration and prioritization rules over time, based on changing customer behavior, seasonality, and other business-specific insights
- Real-time adaptability: IA technologies have limited adaptability, requiring manual updates for rule changes or handling exceptions. If an IA system encounters an unexpected input, it may either fail or escalate for human intervention. Agentic AI, however, autonomously assesses and adjusts to new conditions. It modifies actions, creates new logic, and adapts strategies based on real-time data, all without human intervention. For instance, agentic AI can manage inventory in response to shortages, proactively communicate with suppliers, and re-prioritize orders to adapt to changing demand patterns, learning and improving continuously
Exhibit 2: Comparison between traditional IA and agentic AI
Source: Everest Group (2024)
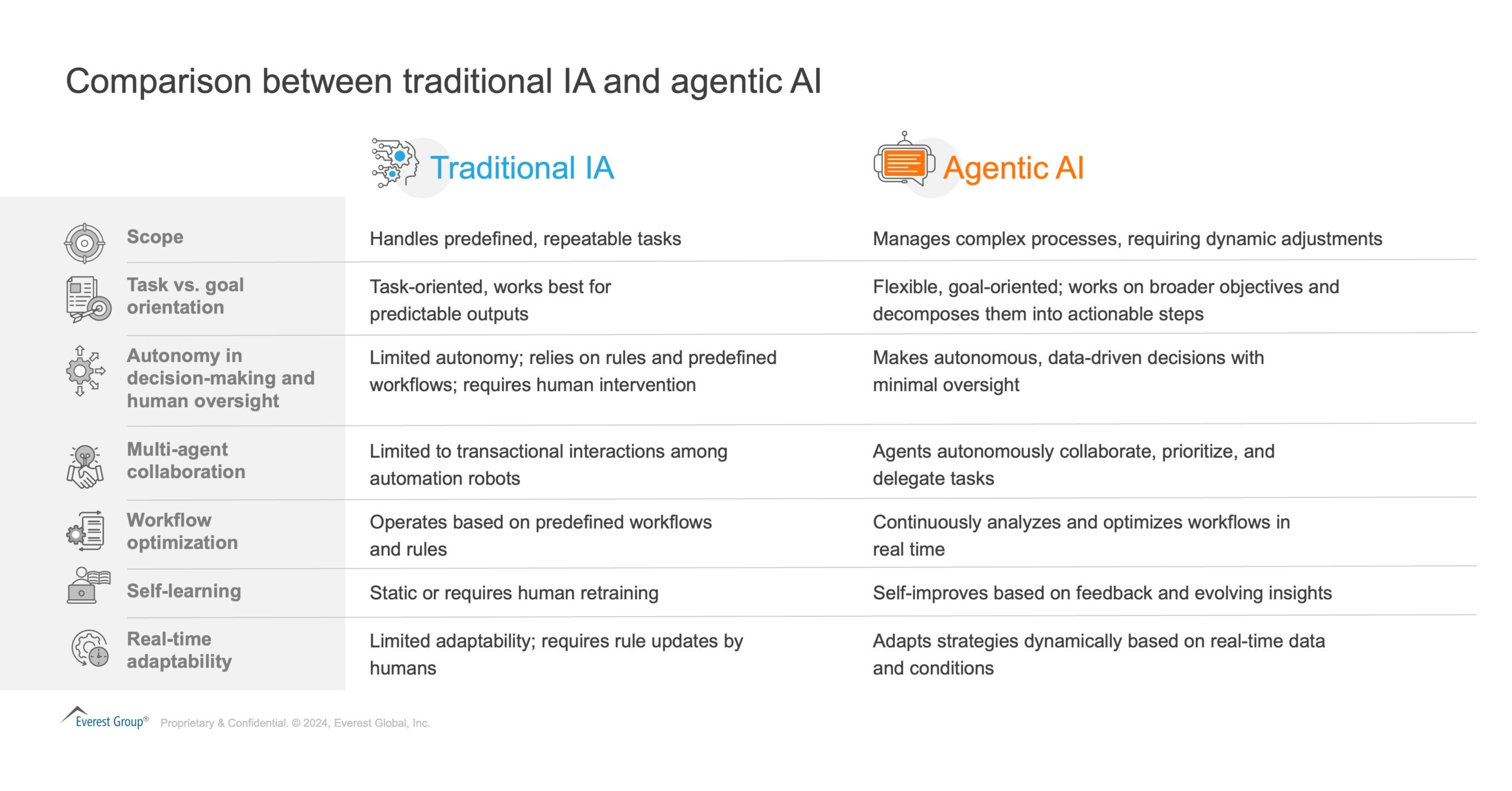
As summarized in Exhibit 2 above, agentic AI surpasses IA in its ability to adapt, learn, and autonomously make decisions. Its broader scope, goal-driven focus, and real-time adaptability enable it to handle complex environments with minimal human oversight, enhancing both operational efficiency and resilience.
Understanding the boundaries of agentic AI
While it is crucial to understand what agentic AI is capable of, it is also important to understand the boundaries in which it functions.
Agentic AI systems show remarkable autonomy and can make decisions based on data, but they are ultimately bound by the parameters set by their programming. They make data-driven decisions within a defined framework. This structured approach ensures that their operations align with specific objectives determined by human developers.
Fundamentally, these systems function as sophisticated tools designed to enhance efficiency and productivity, rather than as sentient beings capable of independent thought or emotional reasoning. They lack the free will or emotional depth that characterizes human decision-making processes, which are influenced by a complex interplay of subjective experiences, values, and social contexts. This distinction is crucial for businesses and users to comprehend, as it helps set realistic expectations regarding the capabilities and limitations of these technologies.
Thus, agentic AI’s decision-making abilities stem from advanced algorithms and data processing techniques – not from any form of independent consciousness. The leap from automation to agentic AI is substantial, but it is still a leap within the confines of human-controlled programming and objectives.
Conclusion
Agentic AI will be crucial for enabling organizations to automate tasks, decisions, and goals. This shift offers operational autonomy but introduces challenges, including the need for strong data governance, orchestration, ethical decision-making frameworks, and defined limits on AI power. Responsible and transparent integration of agentic AI with the surrounding ecosystem is essential for its effective use.
Furthermore, understanding agentic AI deeply is essential for effective industry implementation. The shift from traditional IA to agentic AI-led automation is significant, but it should be viewed as a technology that enhances human capabilities such as decision-making but does not replace human judgment or creativity. Therefore, organizations must evaluate the implications of using agentic AI for critical decisions and assess the context for its deployment.
By acknowledging the boundaries of agentic AI, organizations can leverage its strengths while avoiding potential pitfalls. This approach ensures agentic AI acts as a powerful ally in the digital landscape, empowering businesses to thrive responsibly in a rapidly evolving world.
If you found this blog interesting, check out our Innovation Watch: Agentic AI Products report which delves deeper into another topic worked regarding agentic AI. You may also be interested in our latest blog Navigating the Agentic AI Tech Landscape: Discovering the Ideal Strategic Partner | Blog – Everest Group)
If you have any questions, would like to gain expertise in agentic AI and artificial intelligence, or would like to reach out to discuss these topics in more depth, contact Vaibhav Bansal ([email protected]), Samikshya Meher ([email protected]) and Divya Chandak ([email protected]).