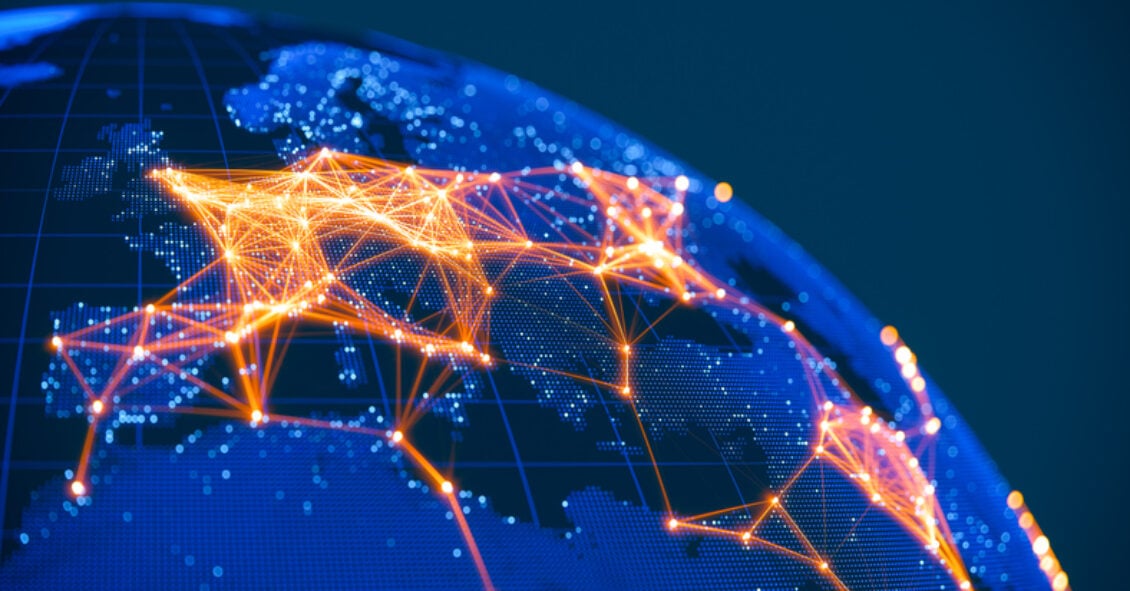
Artificial intelligence (AI) is no longer just an assistant—it’s becoming autonomous. Agentic AI, capable of self-directed decision-making, is poised to revolutionize clinical trials and healthcare delivery.
While everyone is talking about AI, this space is evolving at an incredible pace. What once started as a mere assistant, automating tasks and supporting data analysis – has now grown into a powerful force, transforming industries. One of the most exciting areas of impact? Clinical trials, where AI is revolutionizing how drugs are tested and approved.
Just last year, Generative AI (gen AI) stunned the world with its creative capabilities. But are we done? Not at all. As we enter 2025, a new era of AI is emerging – Agentic AI – a technology designed to act autonomously, make real-time decisions, and adapt dynamically. In clinical trials, this means AI can optimize patient recruitment, enhance trial efficiency, and even predict outcomes with better accuracy.
But wait, there’s more!
Reach out to discuss this topic in depth.
Before diving into Agentic AI, let’s first understand the evolution of AI and how each stage has been distinct in its capabilities and impact.
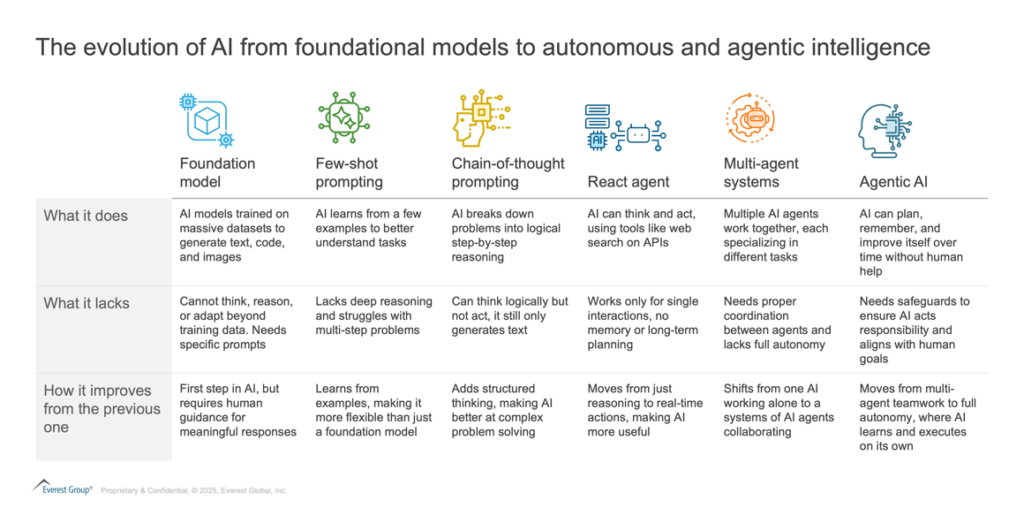
Agentic AI – the next big thing?
Agentic AI is an advanced form of AI that enables autonomous agents with the ability to make decisions, adapt dynamically, and operate independently. These agents can execute using natural language inputs, set their own objectives, develop strategic plans, and take actions to achieve their goals without constant human intervention.
In simple terms, you can think of Agentic AI like a self-driving car – it does not just follow orders; it adapts to road conditions in real time.
Key characteristics of agentic AI include:
- Autonomy: perform tasks independently
- Reasoning: make advanced decisions
- Flexible planning: adjust plans based on prevailing circumstances
- Workflow optimization: efficiently execute multistep, complex processes
- Natural language understanding: comprehend and follow complex instructions
- Continuous improvement: learn from historical data and feedback
- System integration: integrate with diverse enterprise systems
The key to successful Agentic AI lies in training models on diverse datasets, while providing clear and precise instructions.
Is Agentic AI truly beneficial in clinical trials, or is it just a buzzword? Let’s find out.
The clinical trial landscape is evolving rapidly, and Agentic AI is at the forefront of this transformation. Unlike traditional AI, which primarily assisted in data processing, Agentic AI introduces autonomous decision-making, real-time adaptability, and intelligent automation.
By minimizing manual interventions and optimizing critical processes, it is streamlining patient recruitment, protocol management, risk monitoring, and regulatory compliance. With its ability to act independently and adjust dynamically, Agentic AI is not just supporting clinical trials now – it is also redefining them. Here’s how Agentic AI is reshaping key aspects of clinical trials:
- Site and safety monitoring
AI-powered systems can predict potential risks and adverse events, generating real-time alerts and recommending next-best actions. This proactive approach enhances patient safety and ensures timely interventions
- Protocol setup
By autonomously digitizing, validating, and updating protocols based on dynamic requirements, AI streamlines the trial setup process. This reduces manual effort and ensures adherence to evolving regulatory standards
- Patient recruitment
AI-driven analytics help identify eligible participants, forecast recruitment trends, and automate personalized outreach and follow-ups. This leads to faster enrollment and improved patient engagement
- Data management
AI continuously monitors, standardizes, and validates trial data in real time across multiple sources. This ensures data integrity, minimizes errors, and accelerates decision-making throughout the trial lifecycle
- Regulatory submission preparation
Automating data collation and formatting, AI simplifies the creation of regulatory submission reports. This enhances compliance, reduces administrative burden, and speeds up approval timelines
- Predictive modeling
Leveraging historical data, AI predicts patient outcomes, helping researchers refine treatment protocols and study designs in real time. This data-driven approach optimizes trial efficiency and improves success rates
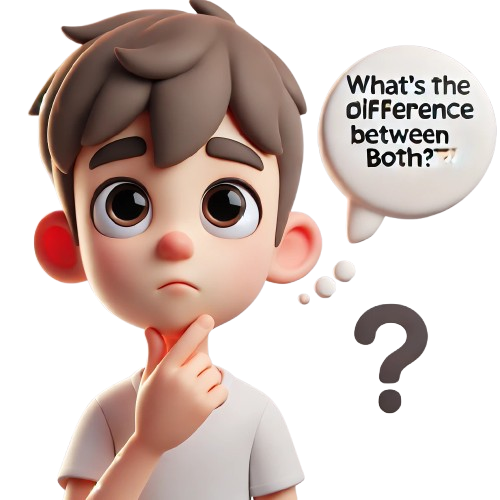
Still not sure about generative AI and Agentic AI? Here’s how they compare in clinical trials
AI is rapidly transforming clinical trials, but with so many advancements, it can be challenging to keep up. What’s the difference between generative AI and agentic AI you may ask? While both enhance efficiency, their roles are quite distinct. Agentic AI is designed for autonomous decision-making, actively managing trial workflows, while generative AI focuses on content creation and data synthesis, making documentation and reporting more seamless. Still confused? Let’s look at the comparison below.
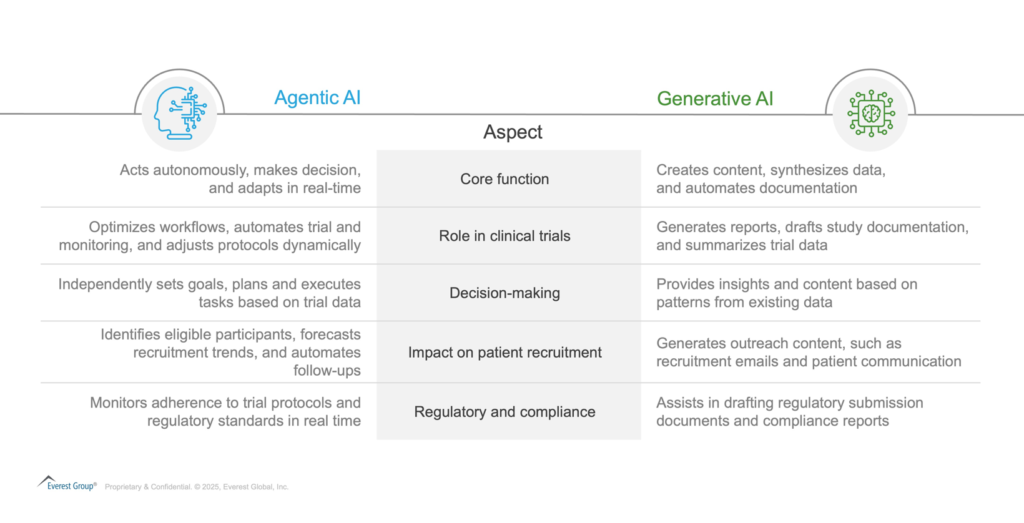
Emerging technologies bring challenges – what does it take for the successful adoption of Agentic AI in clinical trials?
- High quality and standardized data are essential for Agentic AI to generate reliable insights in clinical trials. Inconsistent data sources, such as fragmented Electronic Health Records (EHRs) and lab reports, can lead to biases and inefficiencies. Standardization ensures that AI can process real-world evidence effectively, meeting regulatory expectations and improving trial automation
- Robust Information Technology (IT) infrastructure is necessary for AI to efficiently process vast amounts of clinical trial data. Scalable cloud solutions enable real-time analytics, while secure storage safeguards sensitive patient information. Without a well-built IT infrastructure, AI models may struggle with inefficiencies, cybersecurity risks, and delays in decision-making
- Seamless integration with clinical data systems is crucial for AI to function effectively. It must connect smoothly with existing platforms like EHRs and Clinical Trial Management System (CTMS) to avoid workflow disruptions and redundancies. Adopting modern data exchange frameworks enables AI to interact seamlessly with trial data, leading to faster insights and improved efficiency
- Balancing automation with human oversight is necessary to ensure responsible AI implementation in clinical trials. While AI can optimize workflows and predict trial outcomes, human expertise is essential for handling complex decisions, ensuring ethical compliance, and mitigating errors. A hybrid AI-human model enhances reliability, transparency, and trust
- Ensuring compliance with data and regulatory standards is critical for AI-driven clinical trials. Regulations like General Data Protection Regulation (GDPR) and the Health Insurance Portability and Accountability Act (HIPAA) mandate strict data security and patient safety measures. With a growing emphasis on AI transparency and auditability, strong governance frameworks are needed to ensure AI decisions remain interpretable, compliant, and aligned with ethical standards.
In conclusion, the adoption of Agentic AI in clinical trials is opening new possibilities for improving efficiency, accuracy, and decision-making. While its potential to speed up processes and enhance outcomes is promising, its success depends on thoughtful implementation and responsible oversight.
As the industry moves forward, balancing innovation with careful planning will be key to ensuring AI supports progress in a meaningful way. With the right approach, AI can become a valuable tool in shaping the future of clinical research, making trials more effective and accessible for all.
If you found this blog interesting, check out our Navigating The Agentic AI Tech Landscape: Discovering The Ideal Strategic Partner | Blog – Everest Group, which delves deeper into another topic regarding Agentic AI.
If you have any questions, are interested in exploring Agentic AI and artificial intelligence in clinical trials or would like to discuss these topics in more detail, feel free to Shashwat Bhardawaj ([email protected]) and Nisarg Shah ([email protected]).