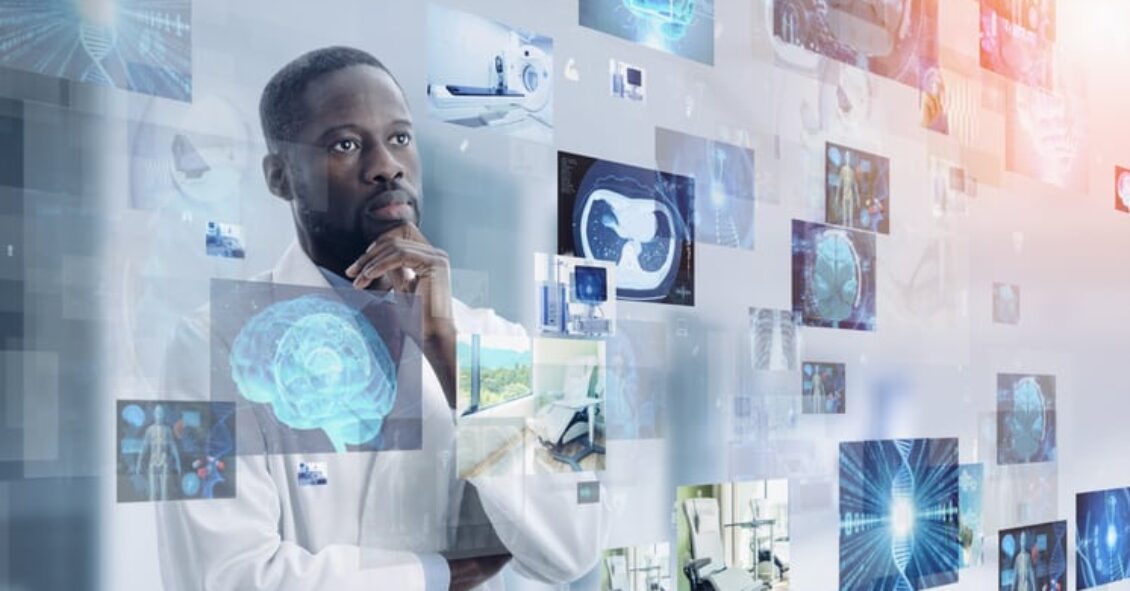
The prospects for Generative AI in clinical development look encouraging. GAI can help speed drug development, improve protocol design, personalize treatment, and bring other benefits. However, the industry must be aware of the risks and responsibly operate GAI. Read on to learn more.
Contact us directly to discuss this topic further.
Given the lengthy, labor-intensive, and expensive process of bringing a new drug to market and conducting a clinical trial, clinical development stakeholders are constantly searching for technological solutions to automate workflows, streamline operations, reduce site and patient burden, and accelerate trial timelines.
Leveraging cutting-edge technology, Generative Artificial Intelligence (GAI) may unlock new horizons and revolutionize the entire clinical development value chain. Let’s explore this further.
GAI works by training underlying large language models (LLMs) on huge datasets containing billions of parameters. For example, ChatGPT is built on GPT-3, a model trained with over 175 billion parameters, far exceeding prior LLMs.
GAI outperforms conventional AI by not only analyzing and interpreting existing data but also generating text, images, audio, video, and other content. The latest innovation, GPT-4, takes this a step further by introducing multi-modality, allowing it to process non-text inputs like images and generate high-quality outputs.
The potential for generative AI in clinical development
In the clinical development field, the opportunities with GAI are simply too big to ignore. It has the power to accelerate drug development, enhance patient engagement, improve protocol design, personalize treatment approaches, generate synthetic data, and much more.
Let’s take a closer look at the potential applications in clinical development to better understand the role that GAI can play in this domain:
- Patient recruitment and screening: By scanning a plethora of health and medical records, GAI can reduce the recruitment funnel and better identify suitable patients for clinical trials. The outcome of these interactions can be fed to a validated digital biomarker for the desired indication. This, in turn, can accelerate patient recruitment and improve diversity ratios
- Synthetic data generation: Synthetic datasets that closely resemble real-world patient data help researchers with limited data or no control group in randomized controlled trials (RCTs). GAI can help conduct simulations, test hypotheses, and accelerate the time to market. With the regulatory push in favor of synthetic control arms, GAI can be instrumental in generating synthetic data
- Protocol authoring: GAI can optimize the process of authoring protocols for clinical trials. It has the ability to scan through vast amounts of scientific literature, past trial histories, and databases and generate insights on the appropriate endpoints, dosage, patient population, treatment arms, and analysis procedures
- Patient engagement: AI-driven by LLMs can analyze patients’ medical history data and preferences and create personalized content. GAI-powered digital avatars can significantly improve patient engagement by providing personalized communication and educational information that ensures patients are included in the process and remain engaged and informed
- Real-time decision-making: During a trial, GAI can continuously monitor patient conditions (through data coming from wearables and sensors) and provide real-time support to investigators and researchers. This may entail preventive interventions, dosage modifications, improving medication adherence, and early detection of adverse events
- Study Data Tabulation Model (SDTM) transformations: SDTM involves mapping clinical data to a standard structure for regulatory submissions. GAI can analyze data from multiple sources and generate mappings that meet SDTM standards. This use of GAI would provide validation and quality controls, and automate repetitive tasks, expediting the whole process
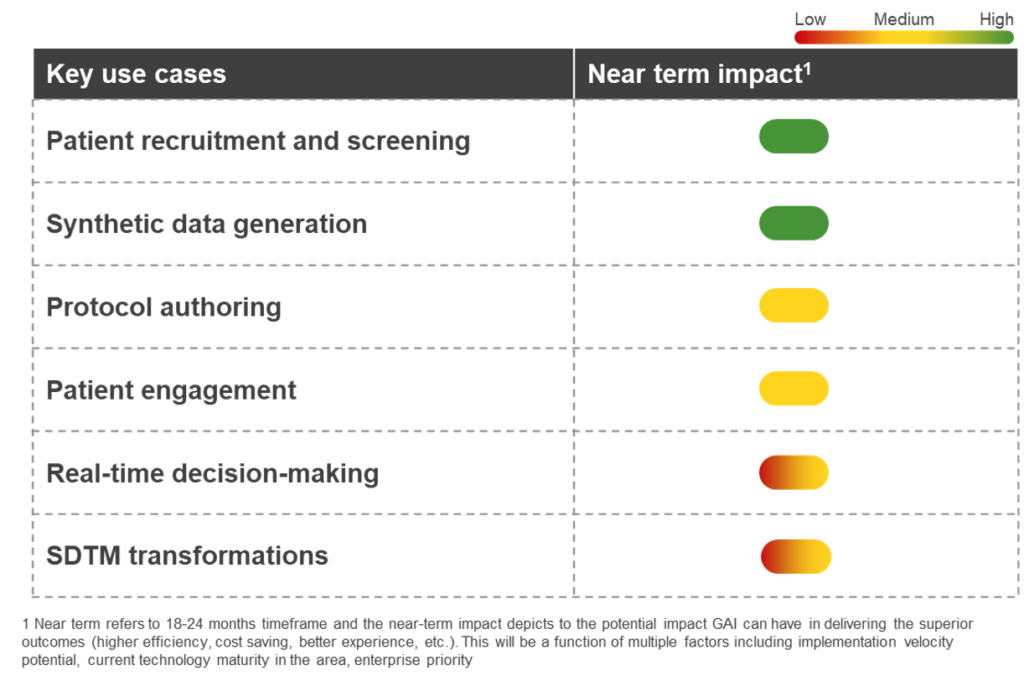
While GAI is generating buzz across industries, like any new technology, its benefits come with challenges. Pharmaceutical enterprises must be aware of the following risks and biases so they can be prepared to address them:
- Data quality and bias: GAI relies on the quality of training data for generating meaningful outcomes. The data used to train the models can have biases that can lead to disparities in patient recruitment and treatment recommendations. New AI models may not be well-suited to handle diverse languages, dialects, and cultures effectively
- Data security and privacy: A crucial consideration for using Generative AI in clinical development is patient data security. Ensuring compliance with the ambiguous regulations for digital technologies and AI in clinical development is complicated. AI models should comply with existing regulations like the Health Insurance Portability and Accountability Act (HIPAA) and the General Data Protection Regulation (GDPR) and be prepared for potential upcoming ones
- Ethical considerations: The use of GAI raises ethical questions, especially in using patient information to train the models. Patient data must be handled with strict confidentiality, and consent must be given at all times before using it to train any model
- Accountability: GAI cannot be held accountable for inaccurate treatment recommendations or a mistake in the protocol design. Completely relying on automated systems raises liability concerns and makes determining accountability challenging
- Sustainability: GAI models are trained on billions of parameters that require extensive computational resources often managed at large-scale data centers. As the use of GAI models and queries continues to grow, the carbon footprint also rises
Over-reliance on AI models without human intervention for decision-making can lead to unwanted consequences. Domain experts, clinicians, and researchers must be present to validate the outputs and use GAI responsibly while having minimal environmental impact.
Technology advances have always brought disruptions, and GAI is no exception. GAI can revolutionize data management processes and become an invaluable tool in clinical development. By better understanding its risks and biases, pharmaceutical enterprises can use GAI responsibility and reap its full benefits – making GAI’s future in clinical development look promising.
To discuss the future of Generative AI in clinical development, contact Anik Dutta, Nisarg Shah, and Madhur Kakade.
Learn more about the use cases and potential of GAI technology in our webinar, Welcoming the AI Summer: How Generative AI is Transforming Experiences.