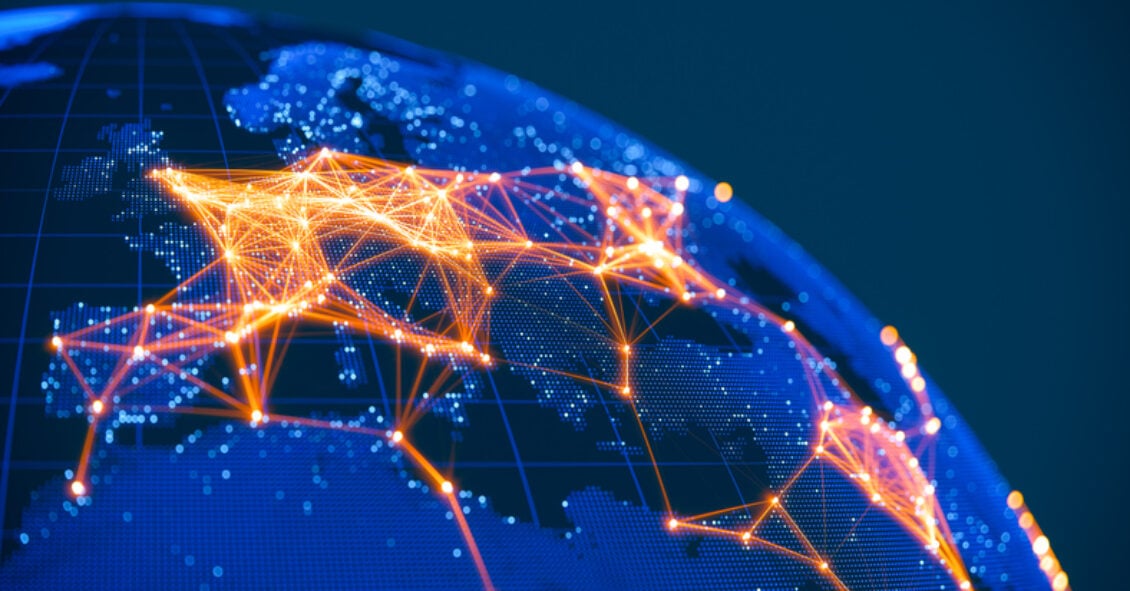
The unseen cost of intelligence: Generative AI’s (gen AI’s) growing environmental footprint
Gen AI is rewriting the rules of innovation, pushing the boundaries of what machines can create, automate, and enhance. But beneath the surface of this technological marvel lies an uncomfortable truth – Artificial Intelligence (AI) is devouring energy, straining water resources, and leaving behind a mounting carbon footprint that can no longer be ignored.
In our previous blogs, we have explored the stakeholder ecosystem for gen AI’s sustainability – technology providers, service providers, and enterprises – and why Diversity, Equity, Inclusion, and Belonging (DEIB) must remain central to its development.
Now, we turn to an issue far more existential – gen AI’s environmental toll and the choices that will determine whether AI is a force for progress or a ticking ecological time bomb.
Reach out to discuss this topic in depth.
The carbon shadow of intelligence
For years, AI’s carbon footprint remained a fringe concern, buried beneath the excitement of technological breakthroughs. But today, the numbers are impossible to ignore.
The World Economic Forum estimates that AI could contribute to between 0.4 to 1.6 gigatons of CO₂ emissions annually by 2035. This means AI’s projected emissions could account for roughly 1% to 4% of current global CO₂ emissions, and with gen AI being an integral part of AI ecosystem, we can expect it to have a significant share in these emissions.
As gen AI becomes more deeply embedded within enterprise workflows, its energy consumption will only accelerate, making its sustainability impact a global issue. At the heart of this crisis is AI’s insatiable appetite for computational power.
Training Large Language Models (LLM’s) requires thousands of high-performance Graphics Processing Units (GPUs), drawing power from grids still dominated by fossil fuels. Inference – the process of running AI models to generate responses – happens millions of times daily, creating a persistent drain on energy resources.
Data centers – the invisible backbone of AI – are expanding at an unprecedented rate, their server farms humming with the raw processing power that fuels everything from AI-driven customer service bots to enterprise automation. But these digital nerve centers are also becoming one of the biggest environmental liabilities of the AI revolution.
A silent drain: AI’s thirst for water
Beyond carbon emissions, AI has another critical, yet often overlooked, environmental cost – water consumption. Gen AI models require vast amounts of water for cooling, ensuring that servers don’t overheat while performing their computationally intensive tasks. But in a world where water scarcity is already an escalating crisis, the location of these data centers is just as important as their energy source.
Take Google’s data center project in Chile, which was expected to consume 7.6 million liters of potable water per day – in a region that has battled severe droughts for years. Public outcry led to a 2024 court ruling suspending the project, highlighting the increasing friction between AI expansion and environmental realities.
The environmental burden extends beyond data centers to the very chips that power AI. The rise in gen AI is driving increased semiconductor demand, and chip fabrication plants (fabs) are notoriously water-intensive, requiring millions of gallons of ultra-pure water for manufacturing processes alone.
The regulatory paradox: Why AI governance struggles to keep pace
As AI advances at breakneck speed, global regulatory efforts are proving insufficient in addressing its environmental and ethical implications. The European Union (EU) AI Act, heralded as a landmark regulation, aims to impose risk-based compliance measures. Yet, its effectiveness remains in question – while the EU tightens AI governance, its ability to curb the industry’s global carbon footprint is limited, as AI infrastructure is largely controlled by tech giants outside its jurisdiction.
This regulatory gap was underscored at the Paris AI Summit 2025, where discussions leaned toward prioritizing innovation over oversight. The prevailing sentiment was that regulations should be ‘light-touch’, in order to avoid stifling competition and innovation. The United States (US) and the United Kingdom (UK) took this stance further, outright refusing to sign a global AI safety declaration. US officials argued that rigid governance would slow innovation, while the UK pushed for voluntary commitments rather than binding laws
The elusive quest to regulate AI’s environmental footprint
Regulating AI emissions is fraught with challenges – no standardized carbon metrics, global cloud distribution, and constant model updates make accountability elusive. Stricter carbon rules could push AI development to less-regulated regions, creating enforcement gaps. Transparency is another hurdle, as AI companies rarely disclose energy usage, limiting oversight. Even where emissions are tracked, regional energy disparities complicate regulation.
Exhibit 1 encompasses the challenges in regulating the environmental footprint of AI.
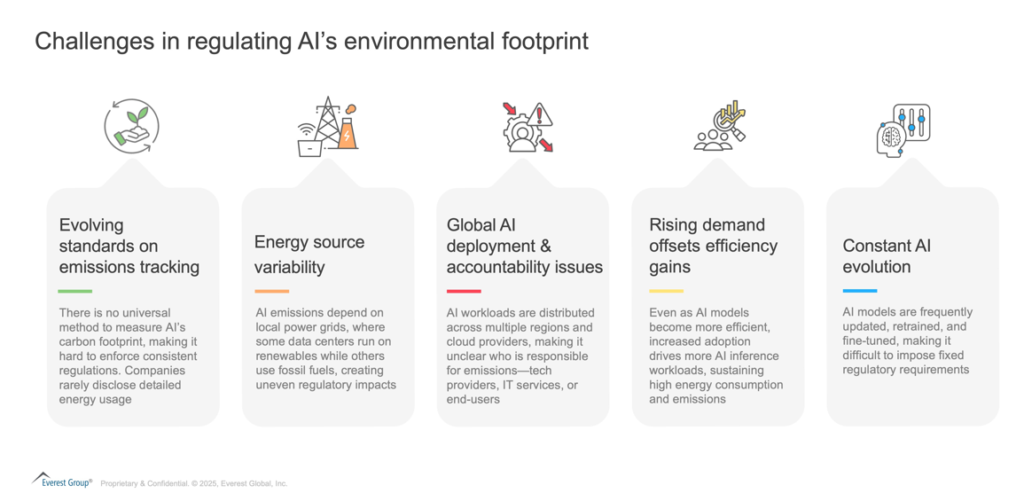
Exhibit 1
While AI models are becoming more efficient, surging demand offsets sustainability gains, ensuring that AI’s carbon footprint continues to grow. Without a unified global approach, AI risks becoming an unchecked environmental burden, despite its promise of technological progress.
A business case for sustainability: Turning green into a competitive edge
If AI continues this trajectory – guzzling power, draining water, and leaving an unchecked carbon footprint – the long-term risks are undeniable. But history has shown that industries that embed sustainability not as an obligation, but as a strategic lever, can redefine their market position.
There’s precedent for voluntary, industry-led sustainability efforts delivering tangible business outcomes. The green data center movement is a prime example – what started as an environmental necessity has now become a strategic differentiator.
Tech giants like Google and Microsoft shifted to renewable energy, developed advanced cooling technologies, and optimized data center efficiency – not just to reduce emissions, but to lower operational costs, mitigate energy price volatility, and enhance brand equity.
Yet, the sustainability advantage extends far beyond infrastructure. The Green Software Foundation and leading tech players are now also championing carbon-aware computing, where AI workloads are dynamically scheduled to run when and where renewable energy is most available.
This not only reduces emissions but also optimizes cloud spend, a growing concern for enterprises scaling AI adoption. Similarly, algorithmic efficiency – through innovations like sparse modeling and quantization – is slashing energy consumption without sacrificing AI performance, giving companies a competitive edge in cost and speed.
The lifecycle of gen AI: Mapping carbon burden and industry responsibility
To fully understand AI’s sustainability challenge, we must break it down into four key stages – each with distinct carbon burdens and clear opportunities for intervention.
1. Model training and development
Carbon burden: Highest
Why: Training massive AI models requires exponentially high energy consumption, often relying on fossil-fuel-powered grids. One Generative Pre-training Transformer (GPT)-3 training cycle emitted 500+ tons of CO₂ – the equivalent to driving a car around the Earth 120 times.
Who’s responsible: Tech providers
What can be done:
- Shift to renewable-powered data centers
- Invest in energy-efficient AI architectures (e.g., sparse models, quantization)
- Disclose energy usage and carbon impact for transparency
2. Deployment and inference
Carbon burden: High
Why: Once trained, AI models will run inference operations millions of times per day , requiring constant computational power. Large-scale AI applications in cloud, chatbots, and automation platforms contribute heavily to energy drain.
Who’s responsible: Service providers
What can be done:
- Optimize AI workloads through more efficient inference models
- Encourage green cloud adoption for enterprise AI use
- Develop AI lifecycle management strategies to retire inefficient models
3. Enterprise adoption and scaling
Carbon burden: Moderate
Why: Companies deploying AI drive demand, often over-provisioning cloud resources and running redundant models. Many lack sustainability benchmarks for AI adoption.
Who’s responsible: Enterprises across industries (e.g., banks, retailers, manufacturers)
What can be done:
- Select AI vendors committed to sustainability
- Optimize AI usage and limit redundant model runs
- Push for greater carbon transparency in AI procurement
4. Model retirement & disposal
Carbon burden: Low
Why: AI models don’t disappear – they require data deletion, storage optimization, and responsible decommissioning to avoid unnecessary cloud waste.
Who’s responsible: All stakeholders – tech providers, service providers, enterprises
What can be done:
- Decommission outdated AI models instead of keeping them active
- Adopt AI-powered energy efficiency tools for monitoring carbon impact
Exhibit 2 depicts the incidence of carbon burden for stakeholders across stages of AI’s lifecycle.
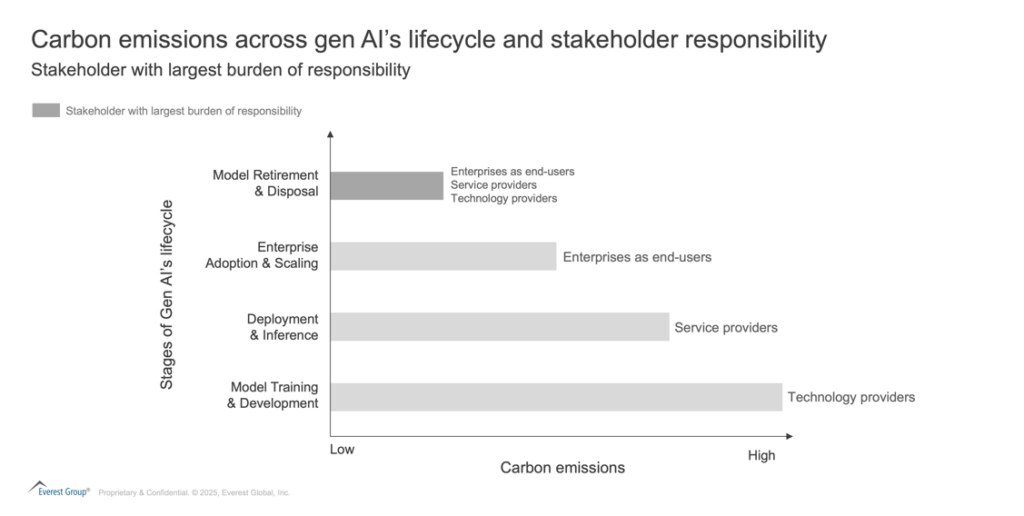
Exhibit 2
A shared responsibility, a shared opportunity
The AI industry stands at a crossroads – one path leads to unchecked environmental destruction, the other to a future where AI and sustainability coexist together.
Some argue that AI’s potential climate solutions outweigh its emissions. The World Economic Forum predicts that AI could drive breakthroughs in climate adaptation – optimizing energy grids, advancing carbon capture, and enhancing disaster prediction models. These innovations could, in theory, outweigh AI’s environmental cost.
But mitigating AI’s own footprint is not optional – it is a necessity. If AI is to be a climate solution rather than part of the problem, its own emissions must be addressed with the same urgency as the problems it seeks to solve.
The question is no longer “Should AI companies reduce their carbon footprint?” but rather “Who will lead the way?”
Sustainability is no longer a regulatory checkbox – it’s a business imperative. Enterprises that bake green principles into their AI strategies aren’t just future-proofing against compliance risks; they’re unlocking operational efficiencies, attracting ESG-conscious investors, and differentiating themselves in an increasingly eco-aware market.
In the race for AI dominance, those who recognize sustainability as an enabler of profitability – not a constraint – will lead the pack.
If you found this blog interesting, check out our Navigating The Agentic AI Tech Landscape: Discovering The Ideal Strategic Partner | Blog – Everest Group, which delves deeper into another topic regarding AI.
If you have any questions or would like to discuss these topics in more detail, feel free to contact Meenakshi Narayanan ([email protected]), Rita Soni ([email protected]), Abhishek Sengupta ([email protected]), Cecilia Van Cauwenberghe ([email protected]) or Kanishka Chakraborty ([email protected]).