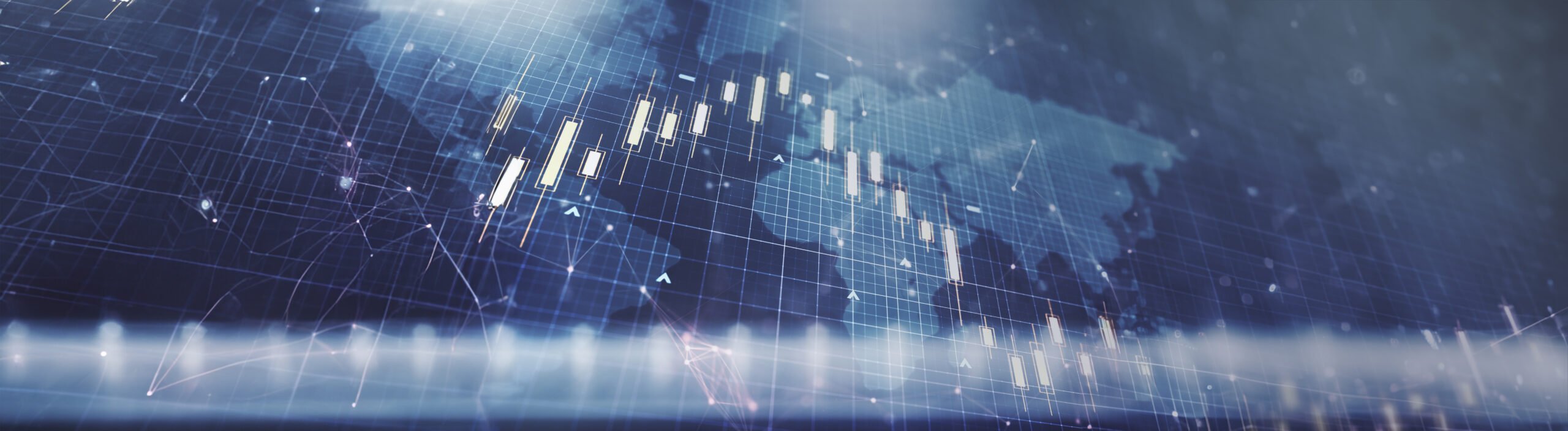
As Artificial Intelligence (AI) continues to revolutionize industries, the demand for robust Information Technology (IT) infrastructure capable of handling immense computational and data processing needs of AI workloads has increased.
While much attention is given to the computational prowess of Graphics Processing Units (GPUs) and Tensor Processing Units (TPUs), networking infrastructure plays an equally pivotal role in enabling seamless data flow and communication within the AI ecosystem.
A high-performance network is not just an enabler; it is the cornerstone of AI success, supporting real-time processing, minimizing bottlenecks, and ensuring ultra-fast data transfer, as our analysts have highlighted below.
Reach out to discuss this topic in depth.
According to our research, 81% of enterprises plan to allocate 50% or more of their infrastructure budget this year to upgrade capabilities specifically for AI. This signals a major market shift that technology providers can capitalize on, as AI deployments become increasingly widespread and sophisticated.
Broadcom’s Chief Executive Officer (CEO), Hock E. Tan, anticipates substantial revenue growth for the company’s AI and AI networking offerings, projecting a revenue of US$60 billion to US$ 90 billion by 2027, a significant leap from the previous estimate of US$15 billion to US$20 billion.
Beyond performance considerations, two major forces are shaping the AI networking landscape:
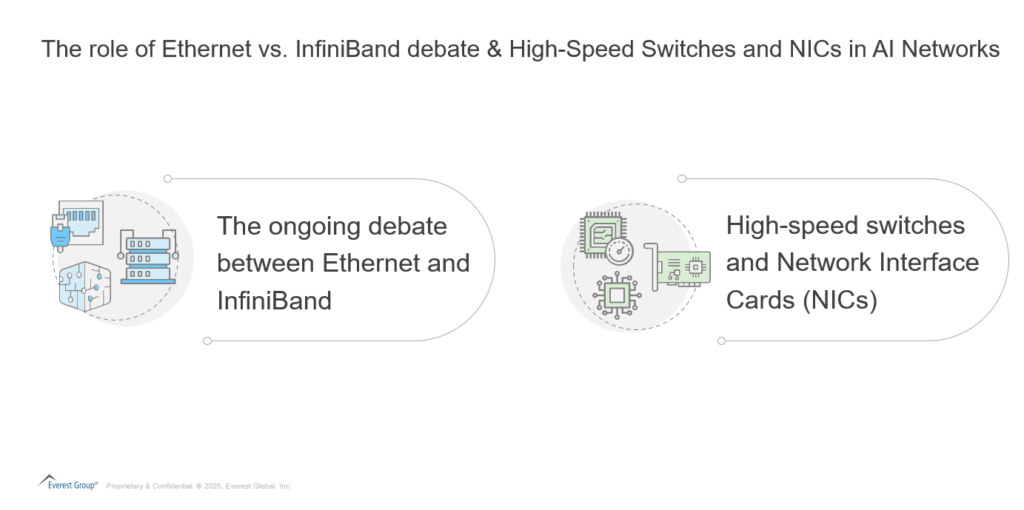
Providers looking to remain competitive must stay ahead of these trends, delivering future-proof, scalable solutions that can adapt to a rapidly evolving AI landscape.
Ethernet or InfiniBand? A strategic choice for AI workloads
A trending question in the AI networking space is whether to adopt Ethernet or InfiniBand. Cisco has long dominated enterprise data centers with Ethernet, while NVIDIA currently leads in delivering high-performance InfiniBand for AI and High-Performance Computing (HPC) environments. This dichotomy forces enterprises to weigh in the benefits of Cisco’s broad Ethernet ecosystem against NVIDIA’s specialized, ultra-low-latency InfiniBand solutions. Both technologies have distinct advantages:
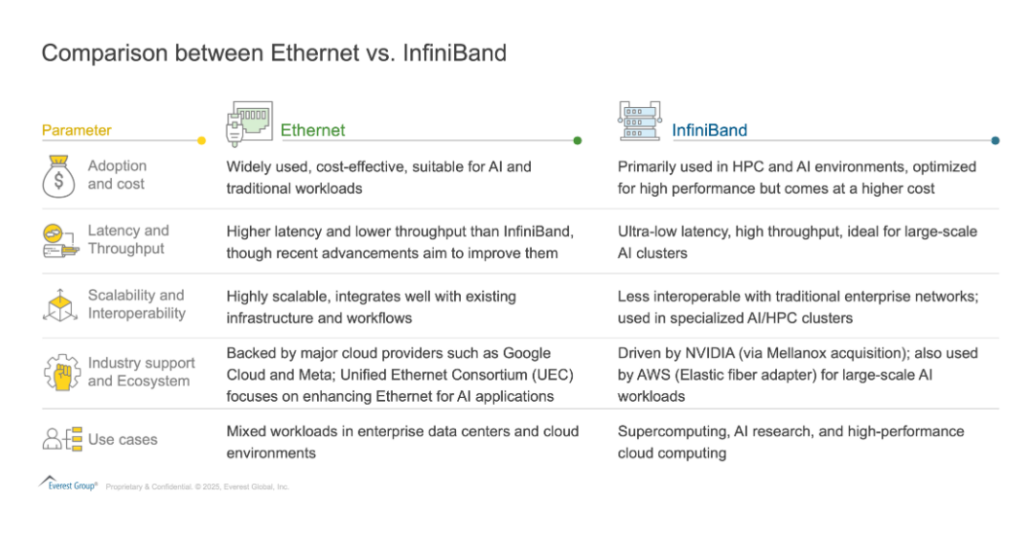
Choosing between these two technologies can be challenging for enterprises.
To capture the best of both technologies, a new trend is emerging: As AI becomes integral to enterprise operations, industry-leading chipmakers, networking vendors, and cloud providers are collaborating to deliver comprehensive platforms spanning raw silicon to high-level application management.
The recent Cisco and NVIDIA partnership exemplifies this shift. By combining Cisco’s advanced networking solutions with NVIDIA’s cutting-edge AI computing capabilities, this collaboration is set to redefine enterprise AI infrastructure. It also signals a bold move, positioning NVIDIA to move beyond HPC and into mainstream data center networking.
The centerpiece of this collaboration is NVIDIA’s Spectrum-X Ethernet platform, which incorporates Cisco’s Silicon One networking chipset. This integration merges the ease of Ethernet deployment with AI-centric features such as adaptive routing, congestion control, and ultra-low latency – benefits traditionally associated with InfiniBand.
For enterprises, this move promises several advantages:
- Reduced complexity through pre-validated, joint solutions
- Accelerated deployments as unified platforms shorten the timeline for AI projects
- Seamless coordination between networking hardware and AI software stacks, enhancing overall performance
The outlook for 2025 and beyond: While Ethernet will continue to improve, InfiniBand currently remains the gold standard for ultra-high performance. Hybrid solutions featuring both technologies are likely to become increasingly common, offering enterprises the flexibility to choose the ideal infrastructure for specific AI workloads. Providers capable of integrating Ethernet and InfiniBand seamlessly will thrive, delivering tailored, cost-effective solutions that meet varied performance requirements.
High-speed switches and Network Interface Cards (NICs): The backbone for AI scalability
AI workloads are no longer confined to single systems. Instead, they span across multiple nodes, racks, and data centers, requiring ultra-high-speed, low-latency interconnects for seamless data exchange. Without the right networking strategy and interconnect solutions, AI models cannot efficiently scale beyond a single system. This shift from isolated AI deployments to highly distributed training and inferencing environments places immense pressure on networking infrastructure to keep pace with escalating data transfer requirements.
As AI applications require ever-faster data processing, network devices such as switches and NICs have evolved significantly. Modern high-speed NICs can now support data transfer speeds of up to 800 Gigabits per second (Gbps), enhancing throughput and reducing latency, both essential for efficient AI model training and inferencing. Similarly, advances in switch technology, including non-blocking architectures and low-latency designs accelerate data flow across networks.
The expanding AI-focused networking market includes established players and innovative newcomers, all striving to stand out through specialized hardware accelerators, software-defined architectures, and innovative System-on-a-Chip (SoC) designs:
- Broadcom introduced 400Gbps NICs to meet the high-bandwidth requirements of AI applications. Its Tomahawk switch series has evolved to support switching capacities up to 51.2 Terabits per second (Tbps)
- Microsoft is investing in Smart NICs, leveraging Fungible’s Internet Protocol (IP), to improve throughput for AI and High-Performance Computing (HPC) workloads
Meanwhile, newer market entrants such as Achronix and Napatech are also gaining traction with Field Programmable Gate Array (FPGA) based NICs and high-speed adapters designed for real-time analytics. Enterprises are adopting these advanced network devices because of benefits such as automation, scalability, energy efficiency, advanced security, and compelling cost-performance ratios. They are making significant investments for AI infrastructure:
- Meta has deployed 800 Gbps NICs to facilitate seamless communication among AI training clusters in its data centers
- Google emphasizes integration between its custom high-speed networking hardware and TensorFlow-based AI frameworks
Despite these breakthroughs, enterprises face challenges such as rising power consumption, cooling requirements, interoperability across multiple vendors, network management complexities, and security concerns in advanced networking components.
Outlook for 2025 and beyond: The AI-driven networking landscape will continue to evolve, with the increasing adoption of ultra-high-speed NICs and advanced switch architectures. While enterprises invest heavily in AI infrastructure, challenges such as power consumption, cooling, and security will necessitate advancements in energy-efficient and interoperable networking solutions. The push for seamless AI model training and real-time analytics will further accelerate demand for intelligent, high-performance networking components.
The path forward for enterprises
As AI-driven initiatives become increasingly pivotal to enterprise success, organizations must invest in high-performance, adaptable networking solutions to fully leverage their AI investments. To achieve optimal outcomes and maintain a competitive edge, consider the following strategic imperatives:
- Adopt modular, scalable solutions: Future-proof data centers with high-speed NICs and switches that can be upgraded as AI workloads evolve. Embrace architectures supporting both Ethernet and InfiniBand to accommodate different use cases
- Plan for multi-vendor interoperability: Avoid vendor lock-in by adopting open standards and ensuring compatibility across various network technologies
- Enhance security, governance, and compliance: As data transfer speeds increase, implement end-to-end encryption and zero-trust architectures to protect sensitive information
- Manage complexity with automation: High-performance networks introduce greater complexity. Invest in automation and monitoring tools that provide real-time visibility into network health and performance
- Control costs with strategic investments: Weigh Total Cost of Ownership (TCO) when deploying new high-performance networking components, factoring in hardware, software, licensing, power, cooling, and ongoing maintenance costs. Consider flexible consumption models to align expenses with actual usage and mitigate budgetary risks
By bringing together these considerations, enterprises can position themselves for success in an AI-driven future where high-speed, low-latency, and energy-efficient networking forms the bedrock of innovation.
Organizations that invest thoughtfully, focusing on open standards, sustainability, and strategic collaboration will capture the transformative potential of AI. Winners in this space will seamlessly integrate advanced networking solutions with sophisticated AI workloads, paving the way for groundbreaking advancements in real-time analytics, autonomous systems, and beyond.
If you found this blog interesting, check out our report, Navigating AI Infrastructure: the Backbone of the AI-Driven Era
If you have any questions, would like to gain expertise in networking infrastructure for AI, or would like to reach out to discuss these topics in more depth, contact Mohit Singhal and Zachariah Chirayil.