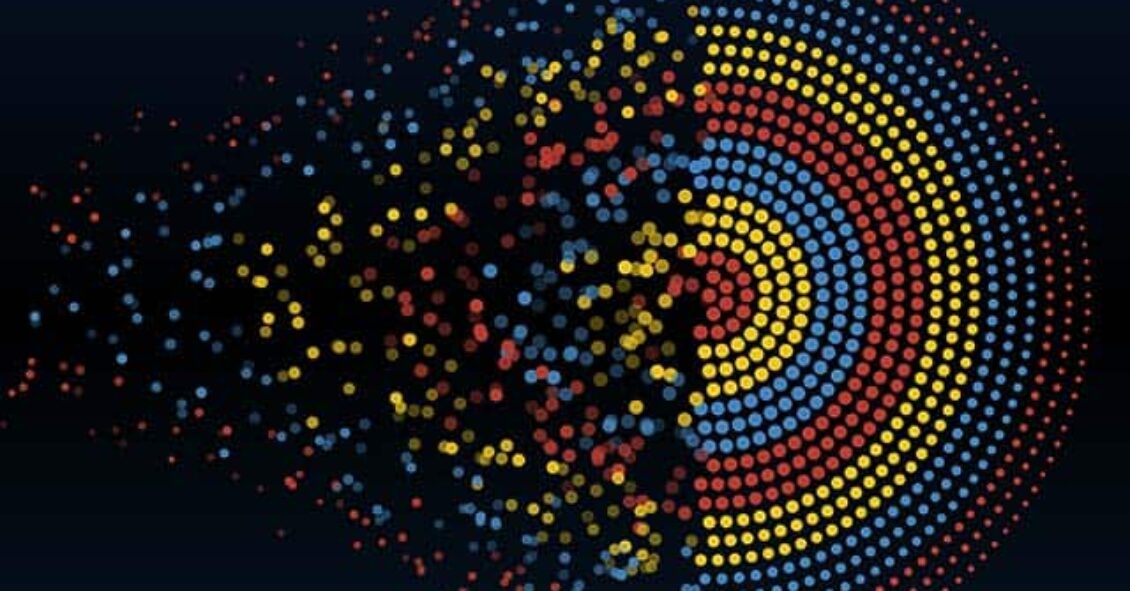
Insight-hungry enterprises are using data storage offerings to better understand consumer behavior and build operational efficiencies. With options expanding from data lakes and warehouses to new combinations such as lakehouses and cloud, how can companies select the best data management solution? We expect technology vendors and hyperscalers to continue to battle it out in the Data and Analytics (D&A) space. Here’s our view on where the market is headed.
Enterprises have been relying on analytics to build operational resilience and agility in business processes. Effective data storage and management are essential to lay down a strong foundation for these analytics use cases.
Major technology vendors operating in the market offer these widely known big data storage product types:
- Data Lakes – a centralized repository that can store data in its raw form at scale
- Data Warehouses – a schema-defined storage offering that can store cleansed and transformed data from different data sources to derive insights
Amazon Web Services, with its Amazon Simple Storage Service (S3), Redshift and Google, with its Google Cloud Storage, and Big Query all cater to enterprises’ varied needs. Other technology vendors such as Cloudera also offer data lake and warehouse solutions as part of their data platform offerings.
The uptick in data migration to the cloud also has led to the rise of cloud data lakes and warehouses. For example, Snowflake’s cloud data lake and warehouse offerings are gaining widespread attention in the market. Its successful IPO in 2020 is also a clear indicator of its success in the cloud data platforms space.
How can enterprises select and customize these offerings based on their requirements? First and foremost, it is essential to understand the varied benefits offered by data lakes and warehouses.
Data Lakes Versus Warehouses
With advancements in Artificial Intelligence (AI) and self-service data preparation tools, cleaning and transforming raw data before analysis is becoming less complex. This is leading to easier implementation of data lakes.
However, as talent scarcity remains a challenge in the data and analytics space, data warehouses emerge as the winner in use cases where business users need to directly derive insights from transformed data without significant technical skills.
Some enterprises also utilize both data lakes and warehouses simultaneously when data is ingested in a data lake and then integrated into a data warehouse for specific BI or reporting use cases. Below, we have outlined some of the other key distinctions between data lakes and warehouses:
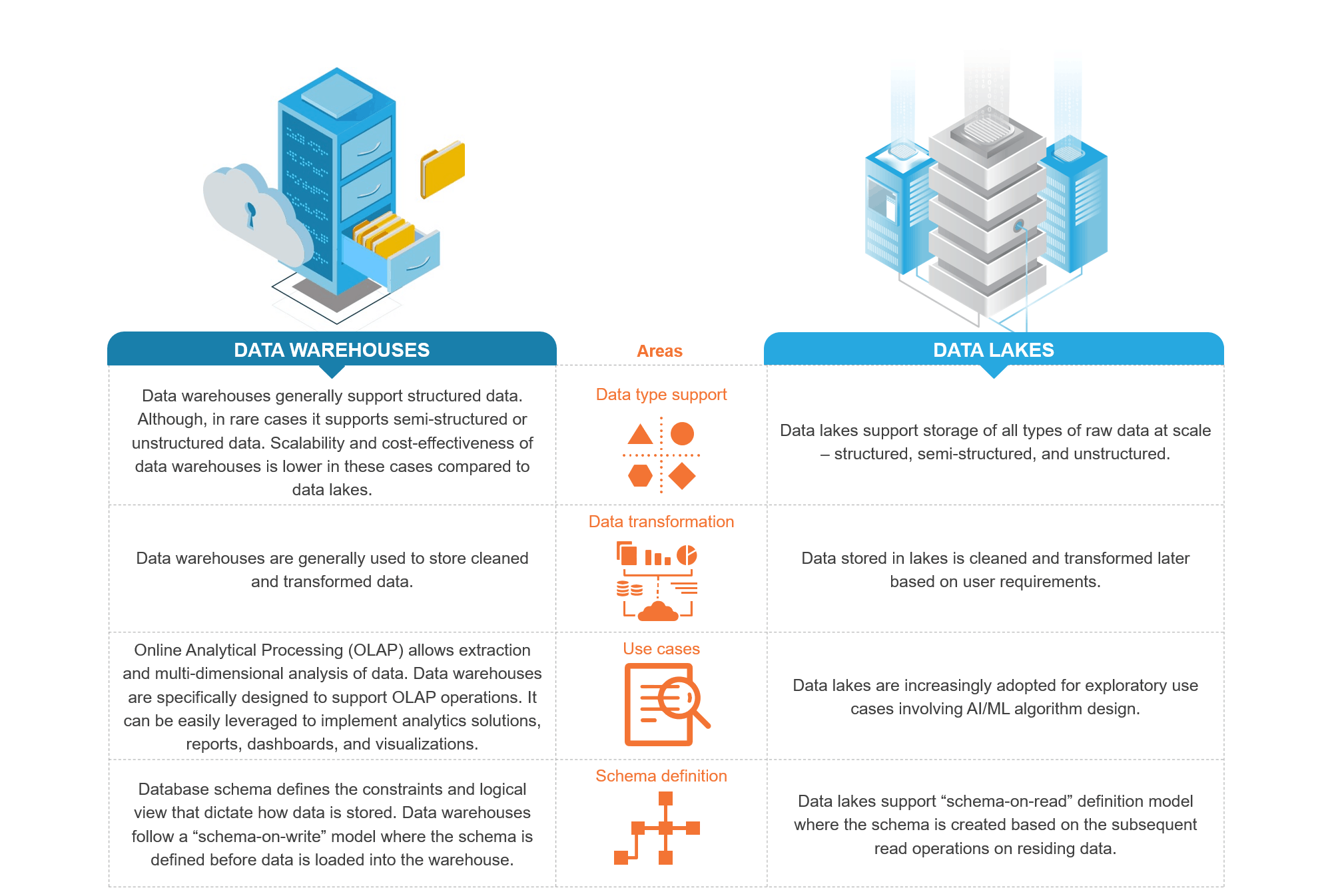
Assessing these differences will help enterprises chalk out what use cases can be built on top of these data stores.
Challenges to Storage Options
Despite the widespread adoption, high storage costs and limitations around using unstructured data for exploratory use cases are key challenges posed by traditional data warehouses. On the other hand, data lakes have limited query management capabilities and high latency due to the absence of in-built query engines. There is also a huge risk of data lakes turning into data swamps due to bad metadata management and governance.
Some niche technology vendors are investing heavily in tools and platforms to tackle some of these challenges. For example, Dremio, a next-generation data lake engine, recently announced a feature that helps users query data directly from data lakes such as AWS S3 through commonly used Business Intelligence (BI) tools such as Power BI or Looker.
The Market Evolution
Contextualizing data stores based on varied business requirements is crucial to the success of any enterprise data strategy. Many enterprises have been successfully implementing data storage offerings to simplify their business processes. The product landscape also has been evolving rapidly due to advancements in cloud, AI, and Extract, Transform, & Load (ETL) tools to cater to the changing enterprise priorities.
Let’s look at some examples where enterprises selected specific data storage offerings to cater to their requirements:
- Nielsen wanted to develop a cloud-based television rating platform that required significant volumes of data to be ingested and processed. It coupled AWS’s data lake and data warehouse offerings and Amazon S3 and Amazon Redshift to store 30 petabytes of data to gather insights around the content that consumers watch. This implementation has enabled Nielsen to measure and analyze more than 30 million households daily. Here, the data lake implementation helped Nielsen manage the huge scale of unstructured data collected from the audience, while the data warehouse implementation helped it to analyze and gather insights from specific datasets
- In 2018, Twitter decided to migrate its data to Google Cloud Platform (GCP) to drive data democratization within the firm. It started leveraging Google’s enterprise data warehouse solution (BigQuery) and big data visualization tool (Data Studio) to enable enterprise-wide analytics and visualization use cases. The data warehouse solution was selected to enable business users with limited technical skills to analyze the data easily
Data lakehouse – a combination of data lake and warehouse – is steadily gaining traction in the market. For example, Wejo, a car data marketplace, implemented Databricks’ data lakehouse architecture that allowed it to directly analyze data of 50 million connected cars stored in data lakes. This has helped the firm provide faster insights to its marketplace customers.
Let us explain what makes a data lakehouse different from a data lake and a warehouse. A data lakehouse supports the implementation of Business Intelligence (BI) and analytics workloads directly on data lakes. It is similar to data warehouses when it comes to the structure and management of data. However, the data is stored in cost-efficient storage units like those used in data lakes. This enables enterprises to access a single data store for both BI and AI/Machine Learning (ML) use cases. With technology vendors and hyperscalers battling it out in the D&A space, emerging offerings including data lakehouses might eventually be the answer to enterprises’ data management woes.
Have you gone forward with the implementation of a data lake, a data warehouse, or both? Have you invested, or are you considering investing in data lakehouses? Please contact [email protected] or [email protected] to share your experiences and priorities while working on these. We’d be happy to connect.