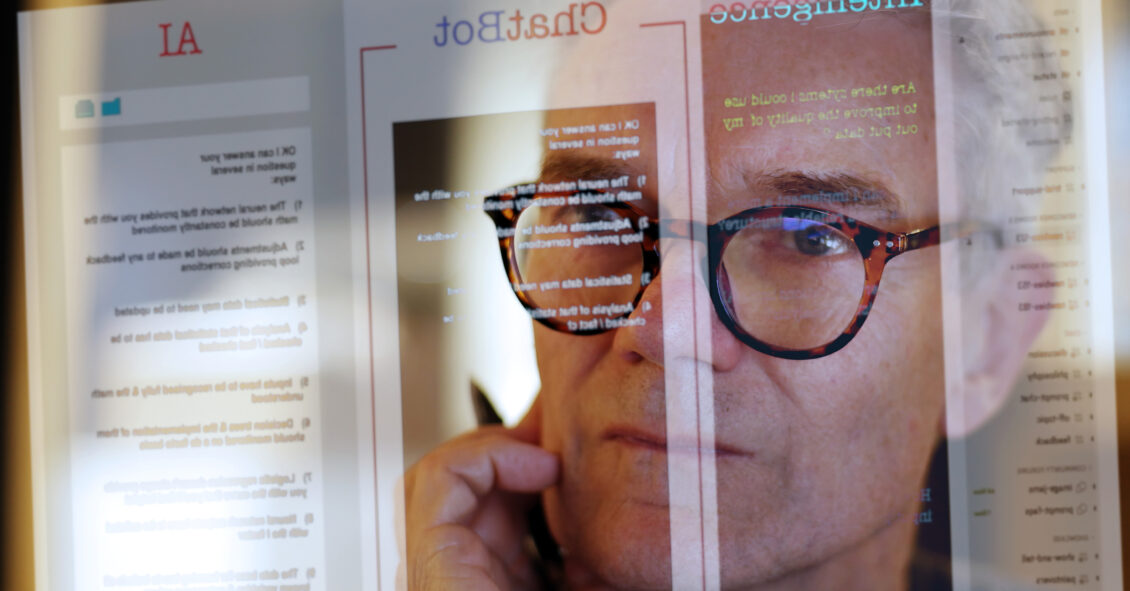
As we stand on the brink of a new technological era, the rise of AI is reshaping our interactions with the digital world.
The rapid proliferation of AI has intensified the demand for scalable, high-performance computing resources, in the process exposing the limitations of traditional infrastructure.
Enterprises are now seeking significant upgrades and expansions to their traditional information technology (IT) infrastructure, in order to keep up with the rising demands of AI workloads.
This has since driven considerable investment into specialized AI infrastructure and tools and services, that can now create the necessary environment for core hardware and infrastructure components to operate at their best.
- According to Everest Group research, 81% of enterprises plan to allocate 50% or more of their infrastructure budget this year to upgrading capabilities specifically for AI
Managing Investments in the Face of Rising AI Demands and Evolving landscape
To accelerate AI development and maintain an edge in the evolving digital landscape, enterprises are increasingly investing in core hardware and infrastructure components. This had led to the surge in demand for high-performance critical computer hardware, networking, and storage infrastructure necessary for AI computations and data management including (Graphics Processing Units) GPUs, (Tensor Processing Units) TPUs, and Virtual Storage Platforms (VSP).
- As per Everest Group research, 46% of enterprises prioritize upgrading computing power such as, graphics processing units (GPUs), central processing units (CPUs), and tensor processing units (TPUs), as one of their top three priorities in AI infrastructure investments
Reach out to discuss this topic in depth.
Providers are now significantly increasing investments to upgrade their supply and secure their positions in a rapidly evolving marketplace.
They are adopting multifaceted strategies to differentiate themselves, secure market share, and address the evolving needs of enterprises for their AI needs. As the market transforms, leading players are making bold strides in the AI arena:
As Nvidia rides the AI wave, AMD battles to disrupt its market dominance in the GPU market
Nvidia, known for its high-end graphics cards for gaming personal computers (PCs), has now crossed US$3 trillion in market cap, owing to the rising demand for its AI chips, critical for advanced AI infrastructure. As Nvidia stands at the forefront of the AI infrastructure market, its GPUs are indispensable for training and deploying sophisticated AI models, including OpenAI’s ChatGPT, leading to its market dominance in the GPU sector.
While Nvidia remains a dominant force in the AI field, other competitors are gradually emerging, aiming to gain market share and driving innovation to break Nvidia’s dominance.
AMD presents a significant challenge to Nvidia in the GPU sector and is working on providing compelling alternatives, particularly for budget-conscious buyers. AMD’s MI300 chip has gained substantial traction amongst startups, as well as with technology giants like Microsoft. It is also constantly investing in this space to bolster its position, as evidenced by its recent multi-billion-dollar acquisition of ZT Systems.
Intel – the computing giant facing challenges, but could that change soon with Gaudi 3?
Intel, traditionally focused on CPUs, has faced challenges in gaining a strong foothold in the GPU market and has been facing stiff competition from competitors, with Nvidia surpassing Intel in annual revenue.
Intel is now intensifying its efforts to close the gap in the AI market. At the recent Intel Vision event, Intel highlighted the forthcoming release of Gaudi 3, an AI accelerator, claiming to be able to outperform Nvidia’s powerful H100 GPU in training large language models (LLMs).
Intel also stated that the Gaudi 3 could deliver similar or even superior performance compared to Nvidia’s H200 for large language model inferencing. Additionally, it claims that Gaudi 3 is focused on reducing energy consumption and has greater power efficiency than the H100, for specific use cases.
Intel’s strategic push to challenge Nvidia’s dominance occurs against a backdrop of persistent shortages in AI accelerator chips, which has created substantial obstacles for tech companies.
Hyperscalers – Nvidia’s largest customers today, potential rivals tomorrow?
Major cloud providers such as Google, Microsoft, Amazon, and Oracle, who together contribute significantly to Nvidia’s revenue, are making a strategic shift toward developing their own processors and in-house chips, to reduce dependency on Nvidia’s GPUs, as well as to drive their own innovation.
Amazon has been rolling out its AI-focused Inferentia and Tranium chips for AI inference and training, offering these through Amazon web services (AWS), as cost-effective alternatives to Nvidia’s products.
Google, a long-time advocate of its Tensor Processing Units (TPUs), recently introduced Trillium, its sixth generation TPU to power its AI models, which it claims is 5 times faster than its predecessor.
Microsoft is also making strides by developing its own AI processors and chips, including the Cobalt 100 CPU, an arm-based processor used for running general purpose computer workloads on the Microsoft Cloud and Maia 100 AI Accelerator.
Emergence of new players and trailblazing startups – Disrupting the AI landscape with innovative approaches?
Several startups are making significant strides within the AI infrastructure landscape, with their innovative approaches.
Cerebras Systems, known for its Wafer-Scale Engine (WSE) designed for high-performance AI workloads, has recently introduced an AI inference service that it claims to be the fastest in the world.
Groq’s Language Processing Unit (LPU) stands out for its high speed in AI inference tasks, offering substantial performance gains for large language models. Groq has also recently raised $640 million for its AI chips.
Groq’s rival SambaNova, has also launched its AI inference platform SambaNova cloud. Similarly other startups like Blaize, an AI chip maker, is developing competitive AI chip technology, with its own unique focus and specialization.
Although Nvidia holds a dominant position, Groq, Cerebras Systems, SambaNova, and other startups are emerging as serious contenders in the marketplace, offering innovative and competitive solutions. It will now be interesting to see how the new players in this space can challenge the technological giants.
Exhibit 1: AI chips and accelerators landscape
How to take the next steps?
As the AI landscape continues to evolve, challenges remain, as enterprise demand for GPUs exceeds supply, leading to a shortage.
This imbalance, combined with high demand, has also driven up GPU prices, making it challenging to find affordable alternatives. As a result, organizations are increasingly exploring alternatives to the dominant players in the AI chip and accelerators market.
But, with so many options, it’s crucial for organizations to carefully evaluate their requirements, budget, and strategic goals, to choose the most suitable options for leveraging AI power effectively. We suggest a two-pronged approach to align organizational AI strategy:
Assess and analyze
Assess your requirements on parameters such as:
- Organizational capabilities and budgetary flexibility: Assess which strategy would suit your budget – purchasing or renting GPUs. Weigh in the initial investment needed, maintenance costs, and long-term operational savings
- AI current workload requirements: Analyze your requirements based on the types of AI workloads and business use-cases (e.g., is your need centered around high-performance training or low latency inference or both)
- Future adaptability: Consider whether your AI workloads may evolve, necessitating reconfigurable hardware or if the efficiency of specialized chips is more important
- Power and space: Assess your organization’s energy efficiency, hardware footprint, and power consumption needs
Align and augment
After the initial assessment and once you have a clear understanding of your AI requirements, develop a roadmap that supports your AI strategy, taking the 5 S into consideration – Scalability, Sustainability, Security, Simplicity, and Stability.
- Ensure your AI strategy is directly aligned with business objectives, such as innovation, operational efficiency, or scaling products, while also being adaptable to future AI workloads
- Augment existing AI infrastructure by partnering with the right vendors that can help you meet your AI workload demands
Exhibit 2: By adopting this two-pronged approach, you can effectively chart the best path to supercharge your AI strategy.
If you found this blog interesting, check out our report, Navigating AI Infrastructure: The Backbone of the AI-Driven Era.
If you have any questions, would like to gain expertise in artificial intelligence, or would like to reach out to discuss these topics in more depth, contact Praharsh Srivastava, Zachariah Chirayil, and Tanvi Rai.