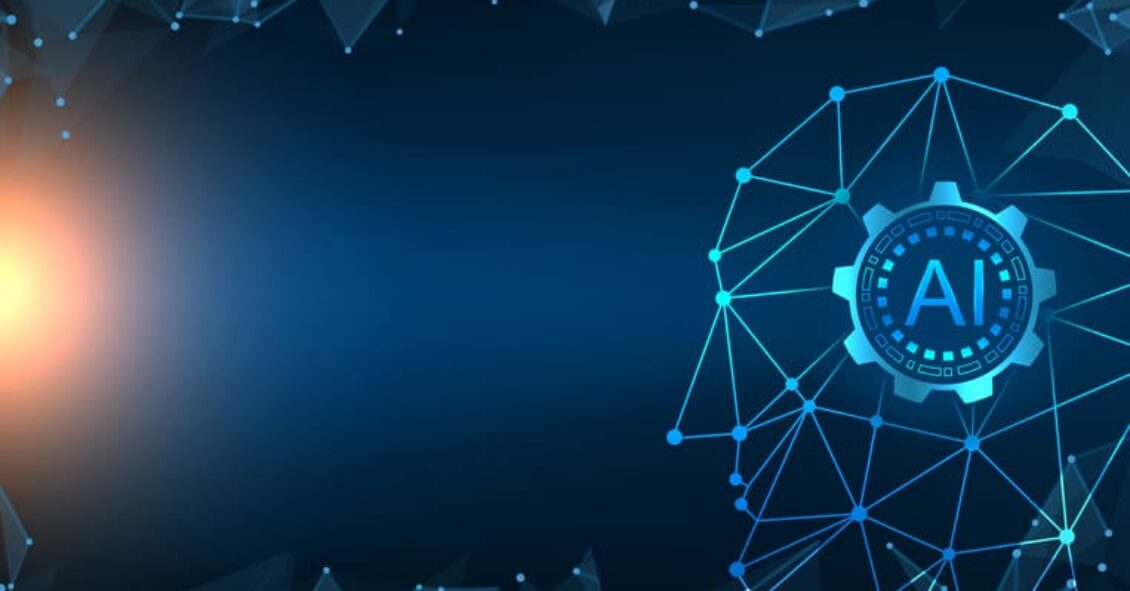
In this era of industrialization for Artificial Intelligence (AI), enterprises are scrambling to embed AI across a plethora of use cases in hopes of achieving higher productivity and enhanced experiences. However, as AI permeates through different functions of an enterprise, managing the entire charter gets tough. Working with multiple Machine Learning (ML) models in both pilot and production can lead to chaos, stretched timelines to market, and stale models. As a result, we see enterprises hamstrung to successfully scale AI enterprise-wide.
MLOps to the rescue
To overcome the challenges enterprises face in their ML journeys and ensure successful industrialization of AI, enterprises need to shift from the current method of model management to a faster and more agile format. An ideal solution that is emerging is MLOps – a confluence of ML and information technology operations based on the concept of DevOps.
According to our recently published primer on MLOps, Successfully Scaling Artificial Intelligence – Machine Learning Operations (MLOps), these sets of practices are aimed at streamlining the ML lifecycle management with enhanced collaboration between data scientists and operations teams. This close partnering accelerates the pace of model development and deployment and helps in managing the entire ML lifecycle.
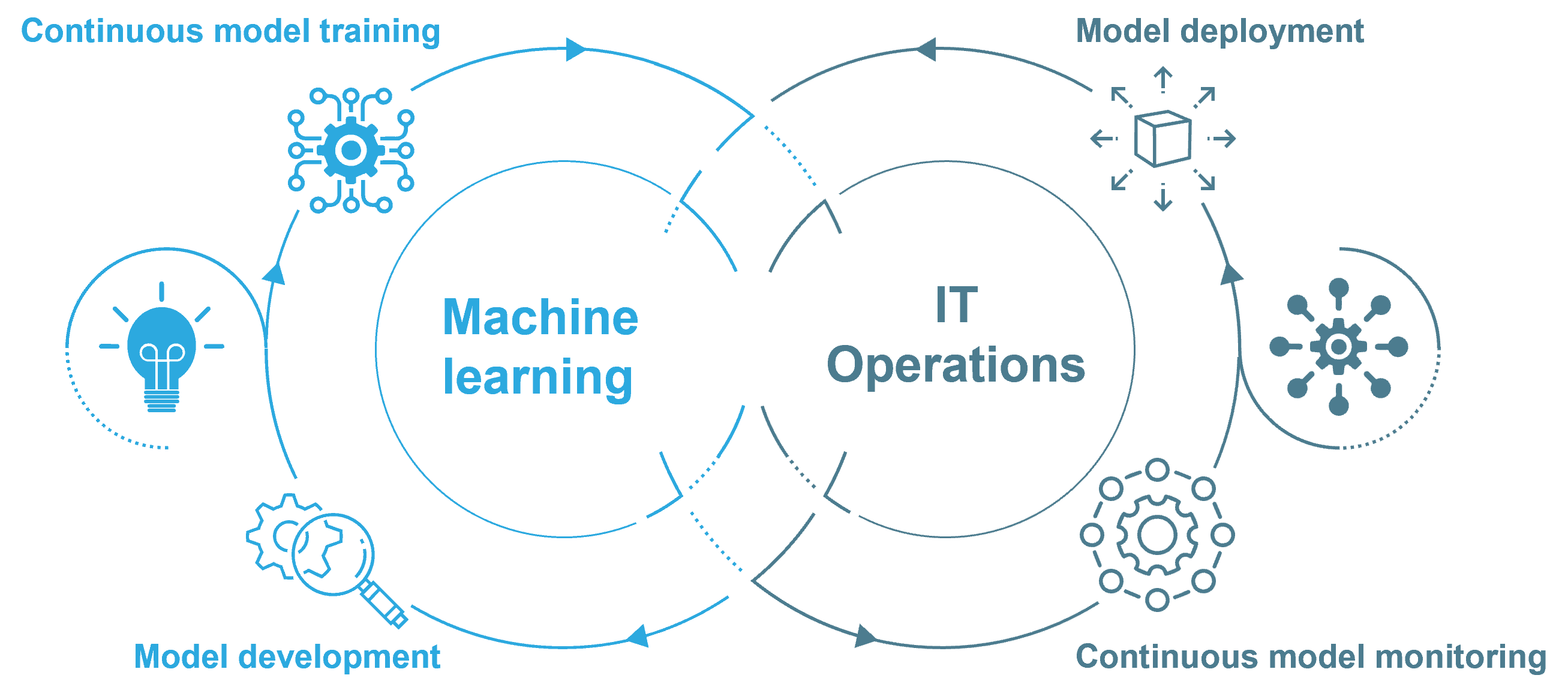
MLOps is modeled on the principles and practices of DevOps. While continuous integration (CI) and continuous delivery (CD) are common to both, MLOps introduces the following two unique concepts:
- Continuous Training (CT): Seeks to automatically and continuously retrain the MLOps models based on incoming data
- Continuous Monitoring (CM): Aims to monitor the performance of the model in terms of its accuracy and drift
We are witnessing MLOps gaining momentum in the ecosystem, with hyperscalers developing dedicated solutions for comprehensive machine learning management to fast-track and simplify the entire process. Just recently, Google launched Vertex AI, a managed AI platform, which aims to solve these precise problems in the form of an end-to-end MLOps solution.
Advantages of using MLOps
MLOps bolsters the scaling of ML models by using a centralized system that assists in logging and tracking the metrics required to maintain thousands of models. Additionally, it helps create repeatable workflows to easily deploy these models.
Below are a few additional benefits of employing MLOps within your enterprise:
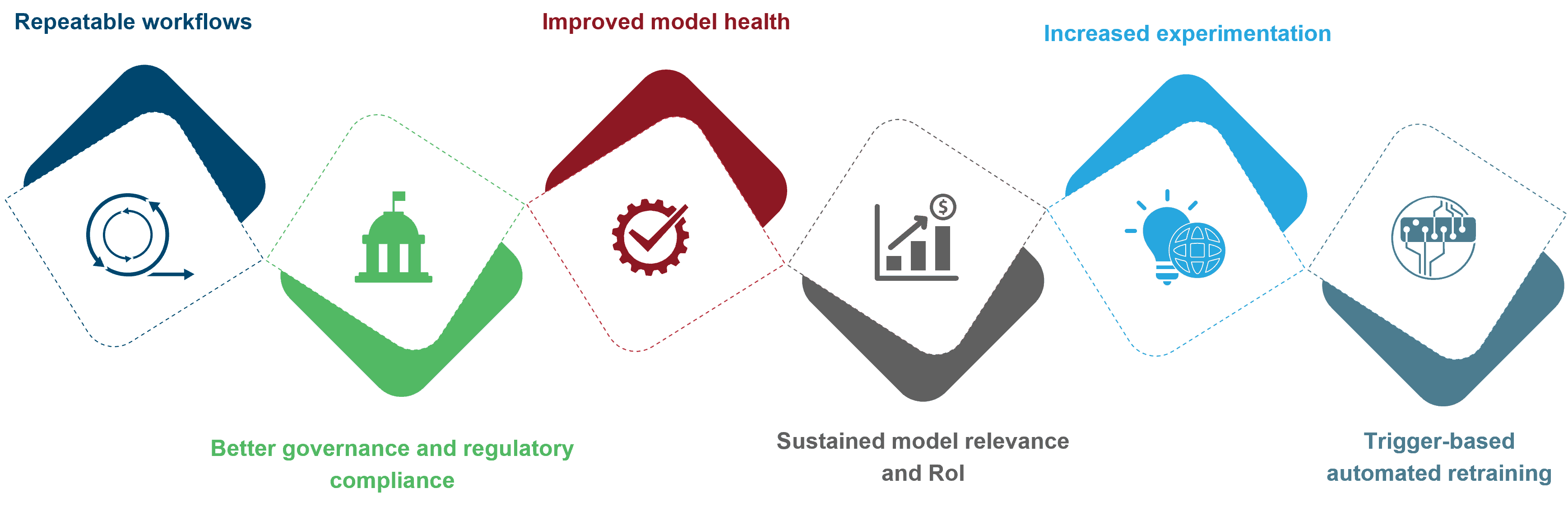
- Repeatable workflows: Saves time and allows data scientists to focus on model building because of the automated workflows for training, testing, and deployment that MLOps provides. It also aids in creating reproducible ML workflows that accelerate fractionalization of the model
- Better governance and regulatory compliance: Simplifies the process of tracking changes made to the model to ensure compliance with regulatory norms for particular industries or geographies
- Improved model health: Helps continuously monitor ML models across different parameters such as accuracy, fairness, biasness, and drift to keep the models in check and ensure they meet thresholds
- Sustained model relevance and RoI: Keeps the model relevant with regular training based on new incoming data so it remains relevant. This helps to keep the model up to date and provide a sustained Return on Investment (RoI)
- Increased experimentation: Spurs experimentation by tracking multiple versions of models trained with different configurations, leading to improved variations
- Trigger-based automated re-training: Helps set up automated re-training of the model based on fresh batches of data or certain triggers such as performance degradation, plateauing or significant drift
Starting your journey with MLOps
Implementing MLOps is complex because it requires a multi-functional and cross-team effort across the key elements of people, process, tools/platforms, and strategy underpinned by rigorous change management.
As enterprises embark on their MLOps journey, here are a few key best practices to pave the way for a smooth transition:
- Build a cross-functional team – Engage team members from the data science, operations, and business front with clearly defined roles to work collaboratively towards a single goal
- Establish common objectives – Set common goals for the cross-functional team to cohesively work toward, realizing that each of the teams that form an MLOps pod may have different and competing objectives
- Construct a modular pipeline – Take a modular approach instead of a monolithic one when building MLOps pipelines since the components built need to be reusable, composable, and shareable across multiple ML pipelines
- Select the right tools and platform – Choose from a plethora of tools that cater to one or more functions (management, modeling, deployment, and monitoring) or from platforms that cater to the end-to-end MLOps value chain
- Set baselines for monitoring – Establish baselines for automated execution of particular actions to increase efficiency and ensure model health in addition to monitoring ML systems
When embarking on the MLOps journey, there is no one-size-fits-all approach. Enterprises need to assess their goals, examine their current ML tooling and talent, and also factor in the available time and resources to arrive at an MLOps strategy that best suits their needs.
For ML to keep pace with the agility of modern business, enterprises need to start experimenting with MLOps now.
Are you looking to scale AI within your enterprise with the help of MLOps? Please share your thoughts with us at [email protected].